Feedforward neural network structure self-organization method based on neuron significance
A network structure and neuron technology, applied in the field of neural network, can solve problems such as network training failure, BP neural network general decline, slow network convergence speed, etc., to improve rationality and scientificity, excellent self-adaptive ability, improve The effect of adaptability
- Summary
- Abstract
- Description
- Claims
- Application Information
AI Technical Summary
Problems solved by technology
Method used
Image
Examples
Embodiment Construction
[0042] The following will clearly and completely describe the technical solutions in the embodiments of the present invention with reference to the accompanying drawings in the embodiments of the present invention. Obviously, the described embodiments are only some, not all, embodiments of the present invention. Based on the embodiments of the present invention, all other embodiments obtained by persons of ordinary skill in the art without making creative efforts belong to the protection scope of the present invention.
[0043] Before introducing the technical scheme of the present invention, introduce some basic knowledge first:
[0044] 1. Introduction of Feedforward neural network
[0045] Feed-forward neural network is one of the most widely used neural network models at present, it can learn and store a large number of input-output pattern mapping relationship, without revealing the mathematical equation describing the mapping relationship in advance. Its basic network s...
PUM
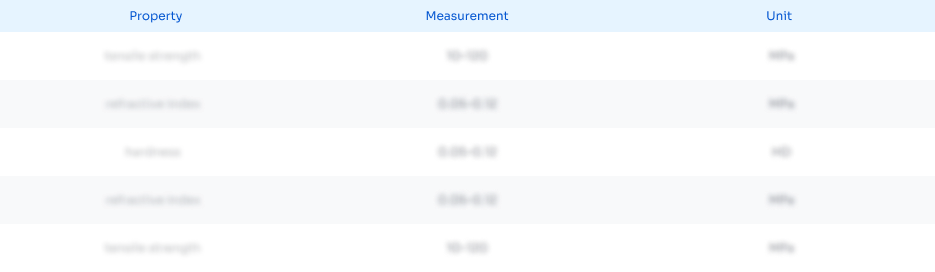
Abstract
Description
Claims
Application Information
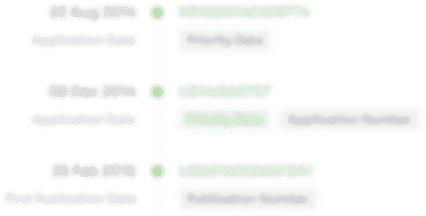
- R&D
- Intellectual Property
- Life Sciences
- Materials
- Tech Scout
- Unparalleled Data Quality
- Higher Quality Content
- 60% Fewer Hallucinations
Browse by: Latest US Patents, China's latest patents, Technical Efficacy Thesaurus, Application Domain, Technology Topic, Popular Technical Reports.
© 2025 PatSnap. All rights reserved.Legal|Privacy policy|Modern Slavery Act Transparency Statement|Sitemap|About US| Contact US: help@patsnap.com