Hyperspectral image low-rank representation clustering method based on bilateral weighting modulation and filtering
A hyperspectral image, low-rank representation technology, applied in the field of remote sensing image processing, can solve the problems of low clustering accuracy, algorithm performance degradation, no effective joint space-spectral information, etc., to achieve high robustness and improve clustering effect Effect
- Summary
- Abstract
- Description
- Claims
- Application Information
AI Technical Summary
Problems solved by technology
Method used
Image
Examples
Embodiment
[0084] combine figure 1 , a hyperspectral image low-rank representation clustering method based on bilateral weighted modulation and filtering, the steps are as follows:
[0085] Step 1, low-rank representation: input a hyperspectral image X 0 ∈R W×H×L , with the image width W=86 shown in Fig. 6 (a), the image height H=83, the Salinas-A data set of the band number L=204 of the image is an experimental example; the original data X 0 Arrange pixel by pixel to form matrix X∈R N×L As the input of the low-rank representation model, N=W×H represents the number of hyperspectral pixels, and the matrix X is used as a self-expression dictionary to establish a low-rank representation minimization model. The model is:
[0086]
[0087] where E∈R N×L is the noise matrix, ||C|| * is the kernel norm of the low-rank representation coefficient matrix C, ||E|| 2,1 is the mixture l of the matrix E 2,1 Norm, λ>0 is a regular parameter; the corresponding low-rank representation coefficie...
PUM
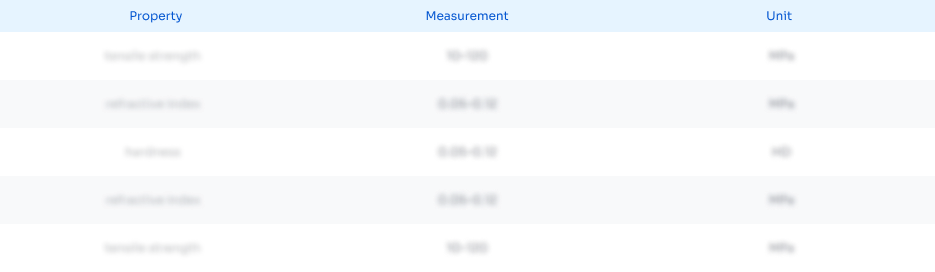
Abstract
Description
Claims
Application Information
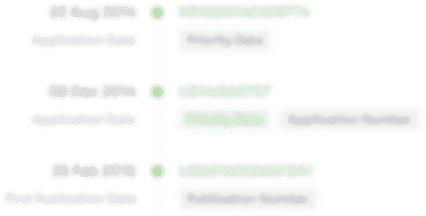
- R&D Engineer
- R&D Manager
- IP Professional
- Industry Leading Data Capabilities
- Powerful AI technology
- Patent DNA Extraction
Browse by: Latest US Patents, China's latest patents, Technical Efficacy Thesaurus, Application Domain, Technology Topic.
© 2024 PatSnap. All rights reserved.Legal|Privacy policy|Modern Slavery Act Transparency Statement|Sitemap