A hyperspectral remote sensing image classification method based on six-layer convolutional neural network and joint spectral-spatial information
A convolutional neural network, hyperspectral remote sensing technology, applied in character and pattern recognition, instruments, computing and other directions, can solve the problems of limited classification effect, high cost, no combination, etc., to improve training and classification speed, reduce training parameters , the effect of improving the training speed
- Summary
- Abstract
- Description
- Claims
- Application Information
AI Technical Summary
Problems solved by technology
Method used
Image
Examples
Embodiment 1
[0046] This example conducts experiments on the Indian Pines dataset, which includes 200 bands, ie U=200, and the image size is 145×145 pixels, ie M=N=145. The hyperspectral remote sensing image is the result of detecting and imaging the area where 16 types of ground objects are distributed, that is, X=16.
[0047] Calculate the energy value of the two-dimensional image corresponding to each band, sort according to the energy value, and extract the first P band data with larger energy value. Because the number of samples in hyperspectral remote sensing images is not uniformly distributed, the number of samples labeled for each category is at most 2455, and the minimum is only 20. Based on 16 types of ground objects, randomly select 10% of hyperspectral remote sensing image pixels as training samples, and the remaining 90% as test samples, and add the selected training samples to 10 categories, as shown in Table 1. In the experiment, the real ground object category and the co...
Embodiment 2
[0054] This example is for the Salinas data set, including 204 bands, ie U=204, and the image size is 512×217 pixels, ie M=512, N=217. The hyperspectral remote sensing image is the result of detecting and imaging the area where 16 types of ground objects are distributed, that is, X=16.
[0055] Calculate the energy value of the two-dimensional image corresponding to each band, sort according to the energy value, and extract the first P band data with larger energy value, Due to the large number of hyperspectral remote sensing image samples, there are 54129 samples, half of which are randomly selected for experiments, and 10% of the samples are randomly selected as training samples, and the remaining 90% are test samples, and the selected training samples The number of categories less than 10 is supplemented to 10. Table 2 shows the real object categories and the corresponding number of training samples and test samples. Randomly remove some training samples and test samples ...
PUM
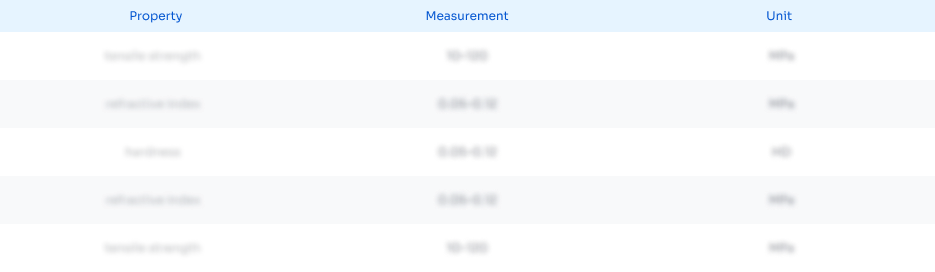
Abstract
Description
Claims
Application Information
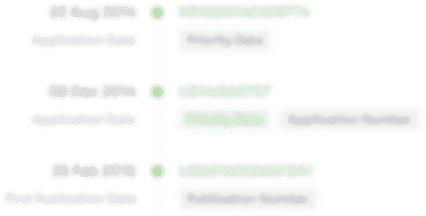
- R&D
- Intellectual Property
- Life Sciences
- Materials
- Tech Scout
- Unparalleled Data Quality
- Higher Quality Content
- 60% Fewer Hallucinations
Browse by: Latest US Patents, China's latest patents, Technical Efficacy Thesaurus, Application Domain, Technology Topic, Popular Technical Reports.
© 2025 PatSnap. All rights reserved.Legal|Privacy policy|Modern Slavery Act Transparency Statement|Sitemap|About US| Contact US: help@patsnap.com