Curvature feature recurrent neural network-based three-dimensional target identification method
A recursive neural network and 3D target technology, applied in the field of 3D target recognition based on the curvature feature recursive neural network, can solve problems such as poor recognition effect, inability to effectively describe 3D target features, and inability to express sequence attributes, etc., to solve image noise problem effect
- Summary
- Abstract
- Description
- Claims
- Application Information
AI Technical Summary
Problems solved by technology
Method used
Image
Examples
Embodiment Construction
[0037] The present invention will be further described in detail below in conjunction with the accompanying drawings and embodiments.
[0038] The present invention is mainly divided into two parts, as figure 1 Shown is the flow chart of the method of the present invention, and the specific implementation process is as follows.
[0039] Step 1: Calculate the joint curvature of the target 3D model, and construct the curvature sketch of the 3D model by extracting the local maximum value of the joint curvature, and use the transmission projection transformation to generate a 360° two-dimensional image as the input of the training recurrent neural network;
[0040] Step 1.1: Set is the normal vector of a given point (x, y, z) on the 3D model. make then p x ,p y ,q x ,q y defined as Then the Gaussian curvature G of the three-dimensional model K for
[0041] G K =|C|,
[0042] where the curvature matrix The mean curvature M of the 3D model K for trace( ) is the t...
PUM
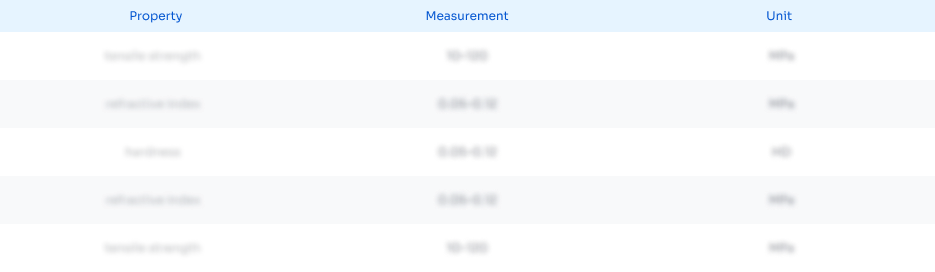
Abstract
Description
Claims
Application Information
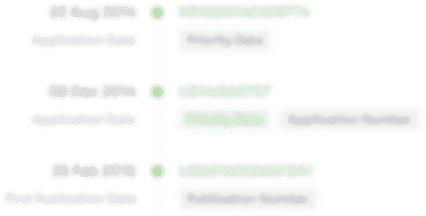
- R&D Engineer
- R&D Manager
- IP Professional
- Industry Leading Data Capabilities
- Powerful AI technology
- Patent DNA Extraction
Browse by: Latest US Patents, China's latest patents, Technical Efficacy Thesaurus, Application Domain, Technology Topic.
© 2024 PatSnap. All rights reserved.Legal|Privacy policy|Modern Slavery Act Transparency Statement|Sitemap