Entity relationship extraction method based on improved deep residual network and attention mechanism
An entity relationship and attention technology, applied in neural learning methods, biological neural network models, instruments, etc., can solve problems such as many feature channels, and achieve the effect of improving noise reduction and performance.
- Summary
- Abstract
- Description
- Claims
- Application Information
AI Technical Summary
Problems solved by technology
Method used
Image
Examples
Embodiment Construction
[0065] The present invention will be further explained below in conjunction with the accompanying drawings and specific embodiments.
[0066] Such as figure 1 As shown, the present invention provides a method for extracting entity relations based on the improved deep residual network and relational word attention mechanism, improves on the basis of the original deep residual network, and proposes P-Gate (segmentation gated residual difference) module and relation word attention mechanism to improve network performance. The P-Gate module mainly improves the deficiencies of the original deep residual network. Combining the characteristics of relationship extraction, the feature channel is segmented by the position of the entity in the sentence, and then the compression operation is performed. On the other hand, the algorithm extracts the relative word information hidden in the sentence in the package and uses it as the attention weight of the sentence. In this way, the noise re...
PUM
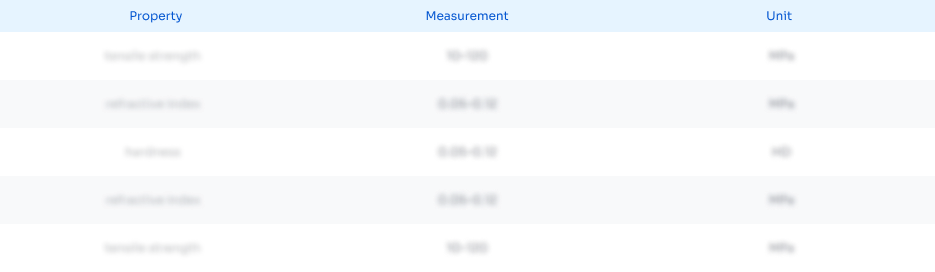
Abstract
Description
Claims
Application Information
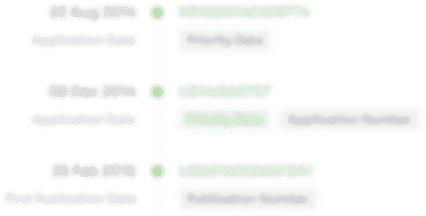
- R&D
- Intellectual Property
- Life Sciences
- Materials
- Tech Scout
- Unparalleled Data Quality
- Higher Quality Content
- 60% Fewer Hallucinations
Browse by: Latest US Patents, China's latest patents, Technical Efficacy Thesaurus, Application Domain, Technology Topic, Popular Technical Reports.
© 2025 PatSnap. All rights reserved.Legal|Privacy policy|Modern Slavery Act Transparency Statement|Sitemap|About US| Contact US: help@patsnap.com