End-to-end three-dimensional human face reconstruction method based on depth neural network
A deep neural network and 3D face technology, applied in biological neural network models, neural architecture, 3D modeling, etc., can solve problems such as recognition and reconstruction effects
- Summary
- Abstract
- Description
- Claims
- Application Information
AI Technical Summary
Problems solved by technology
Method used
Image
Examples
Embodiment Construction
[0028] It should be noted that, in the case of no conflict, the embodiments in the present application and the features in the embodiments can be combined with each other. The present invention will be further described in detail below in conjunction with the drawings and specific embodiments.
[0029] figure 1 It is a system framework diagram of an end-to-end three-dimensional face reconstruction method based on a deep neural network of the present invention. It mainly includes 3D facial shape subspace model, deep neural network (DNN) architecture, and end-to-end training.
[0030] 3D face shape subspace model, which takes a 3D face shape subspace model and treats the 3D face as a linear combination of a set of shapes and a blend shape baseline:
[0031]
[0032] where S is the target 3D face, is the mean face shape, U d is the principal component trained on 3D face scans, α d is the identity parameter vector, U e is the main component of offset training, α e is the...
PUM
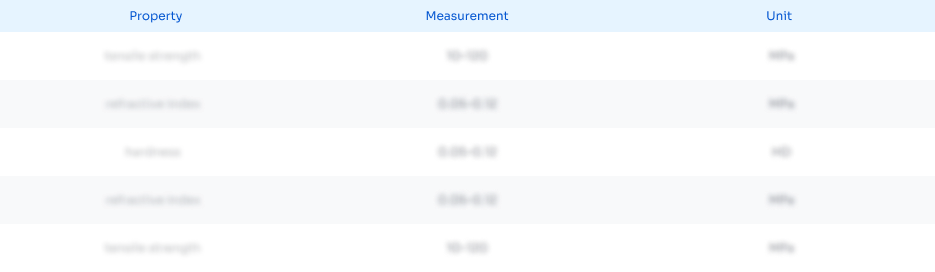
Abstract
Description
Claims
Application Information
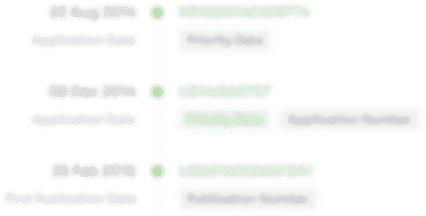
- R&D Engineer
- R&D Manager
- IP Professional
- Industry Leading Data Capabilities
- Powerful AI technology
- Patent DNA Extraction
Browse by: Latest US Patents, China's latest patents, Technical Efficacy Thesaurus, Application Domain, Technology Topic, Popular Technical Reports.
© 2024 PatSnap. All rights reserved.Legal|Privacy policy|Modern Slavery Act Transparency Statement|Sitemap|About US| Contact US: help@patsnap.com