Improved SMOTE re-sampling method for unbalanced data classification
A technology of unbalanced data and data, which is applied in the direction of instruments, character and pattern recognition, computer components, etc., can solve the problems of blindness and marginalization of neighbor selection, reduce overlap between sampling classes, reduce sampling interference, and reduce interference Effect
- Summary
- Abstract
- Description
- Claims
- Application Information
AI Technical Summary
Problems solved by technology
Method used
Image
Examples
Embodiment Construction
[0026] In order to better illustrate the unbalanced data set re-sampling method involved in the present invention, it is applied to the complaint model of Internet TV set-top box users in the following. In this type of model, the data is divided into two categories: the first category is set-top box alarm data; the second category is user complaint data.
[0027] Such as figure 1 The sampling method flow shown, specifically including:
[0028](1) Initialization: Select 10 attributes of the data, and then clean the data. The main goal of cleaning is to remove irrelevant data and redundant information, namely noise samples and unusable data. Data cleaning includes the following two steps: 1. Clean up the wrong data, check the repeatability of the data and mark the samples. The processing of these data is conducive to improving the classification results and avoiding the over-generalization of the data set. 2. Traverse each sample in the entire complaint data set 1, and mark ...
PUM
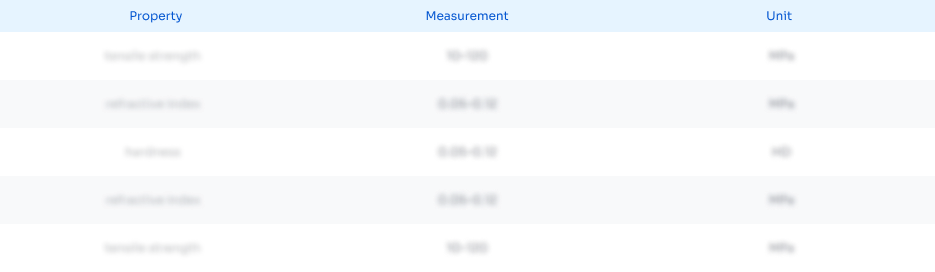
Abstract
Description
Claims
Application Information
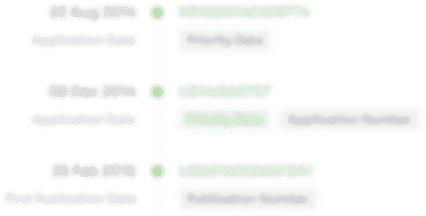
- R&D Engineer
- R&D Manager
- IP Professional
- Industry Leading Data Capabilities
- Powerful AI technology
- Patent DNA Extraction
Browse by: Latest US Patents, China's latest patents, Technical Efficacy Thesaurus, Application Domain, Technology Topic, Popular Technical Reports.
© 2024 PatSnap. All rights reserved.Legal|Privacy policy|Modern Slavery Act Transparency Statement|Sitemap|About US| Contact US: help@patsnap.com