Deep network compression method based on dimensional adaptation Tucker decomposition
A deep network and compression method technology, applied in neural learning methods, biological neural network models, neural architectures, etc., can solve problems such as network compression, waste of storage space, etc., and achieve high compression ratio, effective utilization, The effect of effective storage space
- Summary
- Abstract
- Description
- Claims
- Application Information
AI Technical Summary
Problems solved by technology
Method used
Image
Examples
Embodiment Construction
[0069] Below in conjunction with accompanying drawing, further describe the present invention through embodiment, but do not limit the scope of the present invention in any way.
[0070] The present invention provides a deep network compression method based on dimension adaptive Tucker decomposition, Figure 7 It is a block flow diagram of the deep network compression method of the Tucker decomposition of dimension adaptive adjustment; the flow chart of the deep network compression method of the Tucker decomposition of shared dimension adaptive adjustment is similar to it; the method of the present invention is based on the Tucker decomposition of tensor, aimed at deep network , by adapting and adjusting the size of each dimension of the tensor, a new tensor of any order is generated, and then the tensor decomposition is realized through the learnable kernel tensor and transfer matrix, including the dimension adaptive adjustment process and the weight tensor of dimension adapta...
PUM
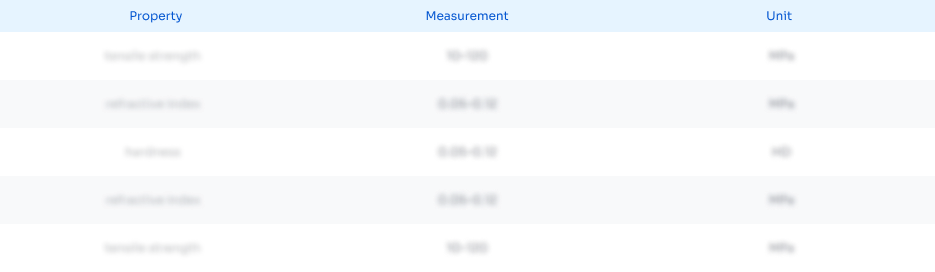
Abstract
Description
Claims
Application Information
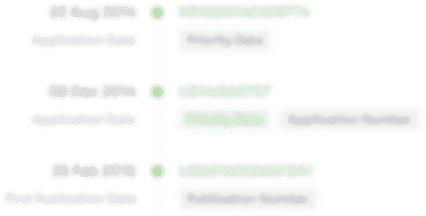
- R&D Engineer
- R&D Manager
- IP Professional
- Industry Leading Data Capabilities
- Powerful AI technology
- Patent DNA Extraction
Browse by: Latest US Patents, China's latest patents, Technical Efficacy Thesaurus, Application Domain, Technology Topic, Popular Technical Reports.
© 2024 PatSnap. All rights reserved.Legal|Privacy policy|Modern Slavery Act Transparency Statement|Sitemap|About US| Contact US: help@patsnap.com