Multi-view learning method of joint low-rank representation (LRR) and sparse regression
A low-rank representation, sparse regression technology, applied in character and pattern recognition, instruments, computer parts, etc., to achieve the effect of improving accuracy
- Summary
- Abstract
- Description
- Claims
- Application Information
AI Technical Summary
Problems solved by technology
Method used
Image
Examples
Embodiment 1
[0031] Studies have shown that image attribute features are very high-level semantic features compared to their original underlying features. To study the visual features of images and predict image memory, the embodiment of the present invention proposes a method for predicting image memory A multi-view learning method that combines low-rank representation and sparse regression, see figure 1 , the method includes the following steps:
[0032] 101: Obtain an image memory data set;
[0033] Among them, the image memorability dataset [1] Contains datasets from SUN [11] 2,222 images of . The memory score of the image is obtained through Amazon Mechanical Turk's Visual Memory Game, and the image memory is a continuous value from 0 to 1. The higher the value, the harder the image is to remember. Sample images with various memory scores such as figure 2 shown.
[0034] 102: Extract low-level features and high-level attribute features from the SUN dataset with image memory sc...
Embodiment 2
[0042] The scheme in embodiment 1 is further introduced below in combination with specific calculation formulas, see the following description for details:
[0043] 201: The Image Memorability Dataset contains 2,222 images from the SUN dataset;
[0044] Wherein, the data set is well known to those skilled in the art, and will not be described in detail in this embodiment of the present invention.
[0045]202: Perform feature extraction on the pictures of the SUN dataset with the image memory score label, the extracted SIFT, Gist, HOG and SSIM features constitute a low-level feature library, and use two types of high-level attribute features, including 327-dimensional scene categories Attributes, 106-dimensional object attributes
[0046] Among them, the above data set includes 2222 pictures in various environments, and each picture is marked with an image memory score, attached figure 2 Shows a sample of images from the database labeled with memory scores. Features express...
Embodiment 3
[0107] Combined with the specific experimental data, Figure 3 to Figure 4 The scheme in embodiment 1 and 2 is carried out feasibility verification, see the following description for details:
[0108] The Image Memorability dataset contains 2,222 images from the SUN dataset. The memory score of the image is obtained through Amazon Mechanical Turk's Visual Memory Game, and the image memory is a continuous value from 0 to 1. The higher the value, the harder the image is to remember, sample images with various memory scores such as figure 2 shown.
[0109] This method adopts two evaluation methods:
[0110] Ranking Correlation Evaluation Method (Ranking Correlation, RC): Get the ranking relationship between the real memory ranking and the predicted memory score, and use the ranking-related Spearman correlation coefficient standard to measure the correlation coefficient between the two rankings. Its value range is [-1,1], and the higher the value, the closer the two sorts are...
PUM
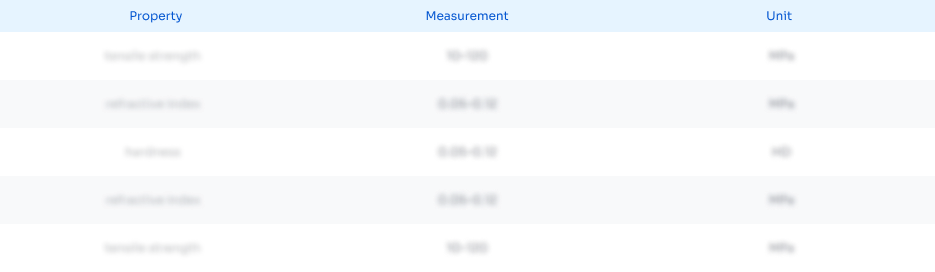
Abstract
Description
Claims
Application Information
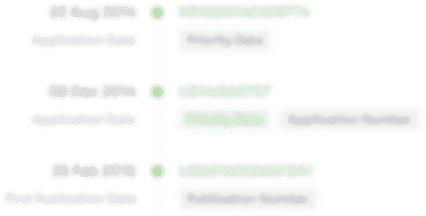
- R&D Engineer
- R&D Manager
- IP Professional
- Industry Leading Data Capabilities
- Powerful AI technology
- Patent DNA Extraction
Browse by: Latest US Patents, China's latest patents, Technical Efficacy Thesaurus, Application Domain, Technology Topic, Popular Technical Reports.
© 2024 PatSnap. All rights reserved.Legal|Privacy policy|Modern Slavery Act Transparency Statement|Sitemap|About US| Contact US: help@patsnap.com