Medical image aided diagnosis and semi-supervised sample generation system
A technology for assisting diagnosis and medical imaging, applied in neural learning methods, biological neural network models, special data processing applications, etc., it can solve the problems of inability to feedback closed-loop, self-learning, and inability to generate high-quality samples, so as to improve labeling efficiency , the effect of improving performance
- Summary
- Abstract
- Description
- Claims
- Application Information
AI Technical Summary
Problems solved by technology
Method used
Image
Examples
Embodiment Construction
[0033] The method of the present invention is further described below in conjunction with accompanying drawing and specific embodiment, and the specific steps of the present embodiment are as follows:
[0034] 1. Design steps
[0035] 1.1. Make training samples and test samples
[0036] 1.1.1. Obtain a large number of CT images: usually, the processed CT data layer thickness is 1.25-3mm, the layer spacing is 0.75-3mm, and the height (y) and width (x) of each layer of CT image are 512×512 pixels, The number of slices (z) of the CT image of a single case is 100-500, and the pixel size in the (z, y, x) direction is 0.5-1.5 mm;
[0037] 1.1.2. Adjust the appropriate HU window level: adjust the original CT image to an appropriate HU window level to eliminate the interference of other organs and tissues in the CT image. For example, when the area of interest is lung tissue, the pixel with the HU value less than -1100 is usually assigned the value -1100, and the pixel with the HU...
PUM
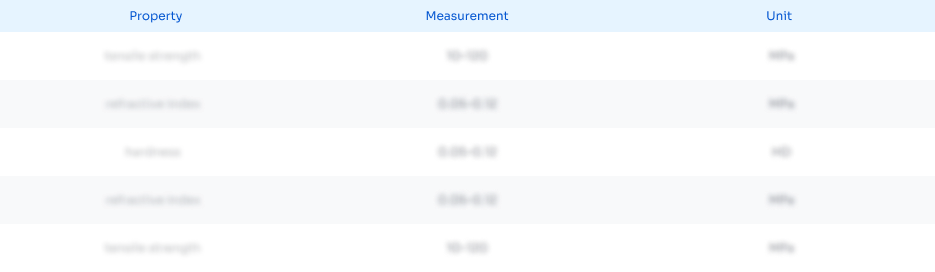
Abstract
Description
Claims
Application Information
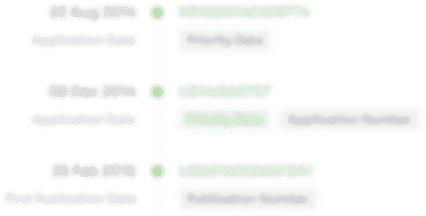
- R&D Engineer
- R&D Manager
- IP Professional
- Industry Leading Data Capabilities
- Powerful AI technology
- Patent DNA Extraction
Browse by: Latest US Patents, China's latest patents, Technical Efficacy Thesaurus, Application Domain, Technology Topic, Popular Technical Reports.
© 2024 PatSnap. All rights reserved.Legal|Privacy policy|Modern Slavery Act Transparency Statement|Sitemap|About US| Contact US: help@patsnap.com