Multi-scale automatic labeling method for images
An automatic labeling, multi-scale technology, applied in the field of machine learning, can solve the problem of labeling that cannot achieve global semantics, and achieve the effect of improving the efficiency of image labeling
- Summary
- Abstract
- Description
- Claims
- Application Information
AI Technical Summary
Problems solved by technology
Method used
Image
Examples
Embodiment Construction
[0016] Such as figure 1 As shown, the multi-scale automatic labeling method for images in this embodiment is characterized in that it includes the following steps:
[0017] Step 1. Find K-nearest neighbor images of the image to be labeled in the training set. The training set includes N images, each image corresponds to several global labels, and each pixel of each image corresponds to a local label.
[0018] In this step, extract the GIST feature vectors of the image to be labeled and all the images in the training set, calculate the Euclidean distance between the GIST feature vector of the image to be labeled and the GIST feature vectors of all the images in the training set, and select K with the smallest Euclidean distance Image, as the K-nearest neighbor image of the image to be labeled. This embodiment extracts GIST feature vectors, in addition, it can also be HOG feature vectors or visual word bag feature vectors.
[0019] Step 2. The frequency of each global label ap...
PUM
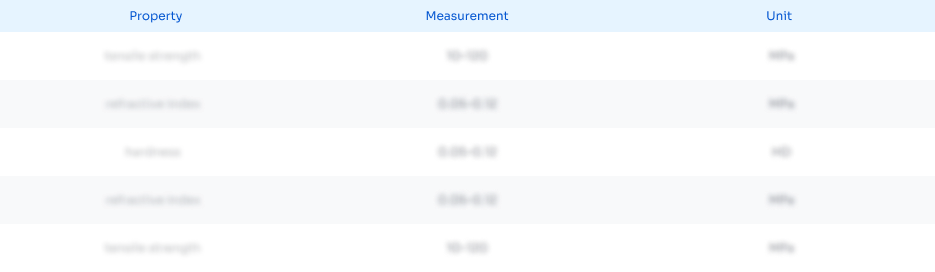
Abstract
Description
Claims
Application Information
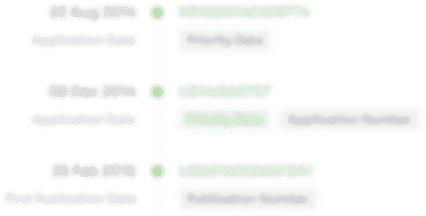
- R&D Engineer
- R&D Manager
- IP Professional
- Industry Leading Data Capabilities
- Powerful AI technology
- Patent DNA Extraction
Browse by: Latest US Patents, China's latest patents, Technical Efficacy Thesaurus, Application Domain, Technology Topic, Popular Technical Reports.
© 2024 PatSnap. All rights reserved.Legal|Privacy policy|Modern Slavery Act Transparency Statement|Sitemap|About US| Contact US: help@patsnap.com