Method for detecting road congestion in combination with visual features and convolutional neural network
A convolutional neural network and visual feature technology, applied in the field of road congestion detection that combines visual features and convolutional neural network, can solve problems such as failure to consider shadow movement prospects, general effect, and inability to fully evaluate road congestion status
- Summary
- Abstract
- Description
- Claims
- Application Information
AI Technical Summary
Problems solved by technology
Method used
Image
Examples
Embodiment Construction
[0057] The present invention will be further described below in conjunction with the accompanying drawings and embodiments.
[0058] like figure 1 As shown, a road congestion detection method that fuses visual features and convolutional neural networks, including:
[0059] Step 1: Use the Gaussian mixture model to perform moving foreground detection and background modeling on the input image video sequence to obtain the background and preliminary moving foreground of the original image;
[0060] Step 2: Input the preliminary moving foreground set into the convolutional neural network to identify moving vehicles, exclude the moving foregrounds of other non-moving vehicles, and obtain the final moving foreground set;
[0061] Step 3: Using the final mobile foreground set to calculate the image visual features reflecting the traffic state, the image visual features include traffic density, traffic speed, traffic occupancy rate and traffic flow;
[0062] Step 4: Calculate the in...
PUM
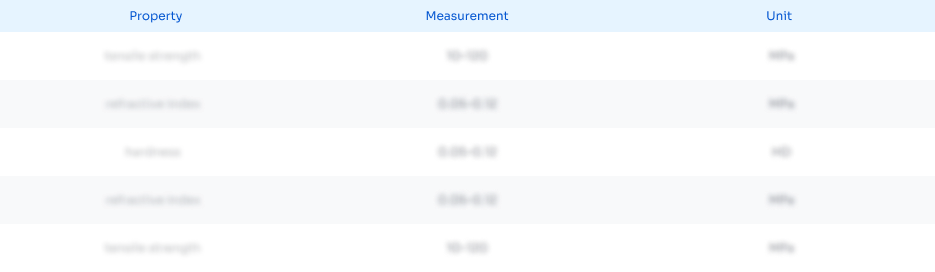
Abstract
Description
Claims
Application Information
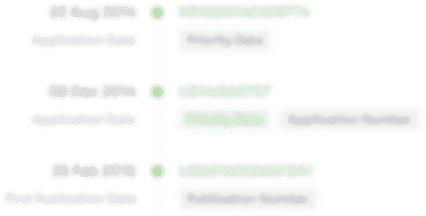
- R&D Engineer
- R&D Manager
- IP Professional
- Industry Leading Data Capabilities
- Powerful AI technology
- Patent DNA Extraction
Browse by: Latest US Patents, China's latest patents, Technical Efficacy Thesaurus, Application Domain, Technology Topic, Popular Technical Reports.
© 2024 PatSnap. All rights reserved.Legal|Privacy policy|Modern Slavery Act Transparency Statement|Sitemap|About US| Contact US: help@patsnap.com