Power transmission line icing thickness predicting method based on LSTM artificial neural network
An artificial neural network and transmission line technology, applied in the field of ice thickness prediction of transmission lines based on LSTM artificial neural network, can solve problems such as hidden dangers to personnel safety, large resource consumption of monitoring equipment, poor reliability of camera equipment, etc., to improve reliability. sexual effect
- Summary
- Abstract
- Description
- Claims
- Application Information
AI Technical Summary
Problems solved by technology
Method used
Image
Examples
Embodiment
[0042] 1) Use the on-line monitoring device to obtain the data of the ice thickness of the transmission line at fixed intervals (such as 2 hours), the monitoring data of the tension terminal set on the transmission tower, the real-time voltage monitoring terminal data and the meteorological terminal monitoring data (temperature, air humidity) , as the basic data of the training sample;
[0043] 2) Use the Lagrange interpolation method to process outliers and missing values of the training sample data. Obtain reliable data that can be input into the LSTM artificial neural network algorithm for calculation, including ice thickness, wire voltage, wire tension, temperature, and air humidity;
[0044] 3) Input the sample data into the LSTM artificial neural network for cyclic recursive calculation until the error value reaches the minimum;
[0045] 4) Use the trained LSTM neural network model as the core algorithm model for predicting the ice thickness of transmission lines in t...
PUM
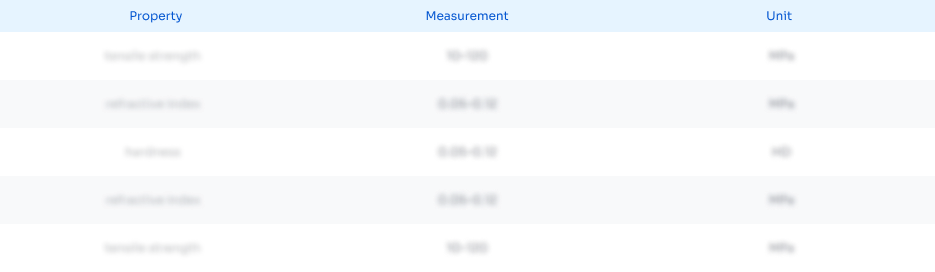
Abstract
Description
Claims
Application Information
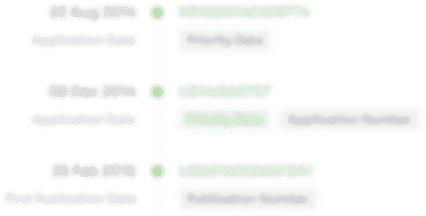
- R&D Engineer
- R&D Manager
- IP Professional
- Industry Leading Data Capabilities
- Powerful AI technology
- Patent DNA Extraction
Browse by: Latest US Patents, China's latest patents, Technical Efficacy Thesaurus, Application Domain, Technology Topic, Popular Technical Reports.
© 2024 PatSnap. All rights reserved.Legal|Privacy policy|Modern Slavery Act Transparency Statement|Sitemap|About US| Contact US: help@patsnap.com