Electrocardiogram diagnosis method and system based on deep convolution neural network
A technology of deep convolution and diagnostic methods, which is applied in the directions of diagnosis, diagnostic recording/measurement, medical science, etc., can solve a large number of problems that cannot be practically solved in large-scale applications, and the accuracy of ECG automatic analysis is difficult to achieve high, so as to reduce the impact Effect
- Summary
- Abstract
- Description
- Claims
- Application Information
AI Technical Summary
Problems solved by technology
Method used
Image
Examples
specific Embodiment approach
[0117] Among them, such as Figure 8 As shown, a specific implementation manner of step S502 includes the following steps:
[0118] S5021, acquiring a training database;
[0119] In the present invention, the training database can be a single sample database, or a plurality of different sample databases can be selected as the training database, such as MIT-BIH arrhythmia database, AHA arrhythmia database, MIT-BIH normal sinus heart rate database etc. The selection of the training database does not affect the design and implementation of the deep convolutional neural network in the present invention, but the categories that the present invention can recognize depend on the categories contained in the training samples.
[0120] S5022, extracting all heart beat positions in the training database;
[0121] The extraction method can adopt the method in the prior art, so it will not be repeated here.
[0122] S5023. Perform preprocessing on all heartbeat positions to obtain a pl...
PUM
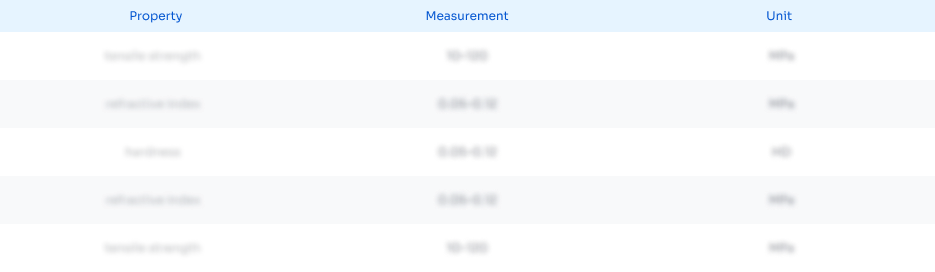
Abstract
Description
Claims
Application Information
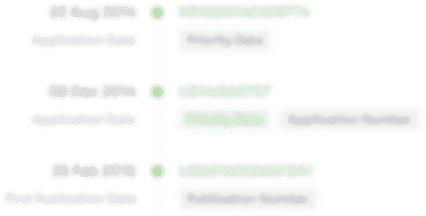
- R&D Engineer
- R&D Manager
- IP Professional
- Industry Leading Data Capabilities
- Powerful AI technology
- Patent DNA Extraction
Browse by: Latest US Patents, China's latest patents, Technical Efficacy Thesaurus, Application Domain, Technology Topic, Popular Technical Reports.
© 2024 PatSnap. All rights reserved.Legal|Privacy policy|Modern Slavery Act Transparency Statement|Sitemap|About US| Contact US: help@patsnap.com