Image memorizing degree predication method based on sparse low-rank regression model
A technology of regression model and prediction method, applied in character and pattern recognition, complex mathematical operations, instruments, etc., to achieve the effect of reducing feature redundancy
- Summary
- Abstract
- Description
- Claims
- Application Information
AI Technical Summary
Problems solved by technology
Method used
Image
Examples
Embodiment 1
[0032] An image memory prediction method based on sparse low-rank regression model, see figure 1 , the method includes the following steps:
[0033] 101: Divide the advanced semantic feature library into an advanced semantic feature library training set and an advanced semantic feature library test set, and respectively extract image memory scores corresponding to the advanced semantic feature library training set and the advanced semantic feature library test set;
[0034] 102: Taking the training set of the advanced semantic feature library and its corresponding image memory score as the learning object, using the alternate iterative method based on the augmented Lagrangian multiplier method to solve it, and training it under the algorithm framework of the sparse low-rank regression model to obtain A relationship model between high-level semantic features and image memory scores;
[0035] 103: Predict the image memory score of the advanced semantic feature library test set ...
Embodiment 2
[0046] The following is combined with specific examples, calculation formulas, figure 2 The scheme in Example 1 is further introduced, see the following description for details:
[0047] 201: Obtain a database of image memory scores;
[0048] The image memory score database used in this method is from the SUN dataset [5] 2222 images were selected as the database images, and the image memory scores corresponding to the images were obtained through the Amazon Mechanical Turk Visual Memory Game (VisualMemory Game) to form the image memory score database. A total of 665 people participated in this game. The image memory score is a continuous value from 0 to 1. The higher the value, the easier the image is to remember. Examples of database images marked with image memory scores figure 2 shown.
[0049] 202: Extract the underlying features and label information of the images in the database to form the underlying feature database and label information database respectively; ...
Embodiment 3
[0094] Combined with the specific experimental data, Figure 2 to Figure 4 , carry out feasibility verification to the scheme in embodiment 1 and 2, see the following description for details:
[0095] 1. Image memory score database:
[0096] The Image Memorability Score database used in this method contains 2222 images from the SUN dataset, which records everyday events and scenes. The memory score of the image was obtained by Amazon Mechanical Turk's Visual Memory Game (Visual Memory Game).
[0097] Two, five comparison methods;
[0098] In this experiment, this method is compared with the following five methods:
[0099] LR (Liner Regression): Use the linear prediction function to train the relationship between the underlying features and the memory score;
[0100] SVR (Support Vector Regression): support vector regression, which strings the underlying features together, and combines the RBF kernel function to learn nonlinear functions to predict image memory;
[0101]R...
PUM
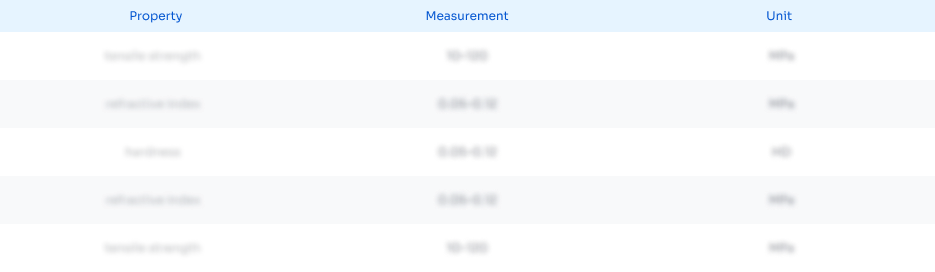
Abstract
Description
Claims
Application Information
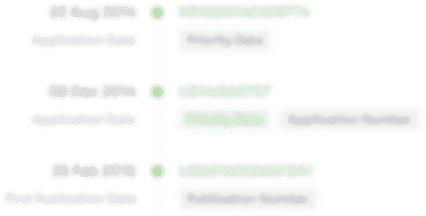
- R&D Engineer
- R&D Manager
- IP Professional
- Industry Leading Data Capabilities
- Powerful AI technology
- Patent DNA Extraction
Browse by: Latest US Patents, China's latest patents, Technical Efficacy Thesaurus, Application Domain, Technology Topic, Popular Technical Reports.
© 2024 PatSnap. All rights reserved.Legal|Privacy policy|Modern Slavery Act Transparency Statement|Sitemap|About US| Contact US: help@patsnap.com