Common spatial pattern and deep learning method based on brain-computer interface auxiliary rehabilitation therapy
A co-spatial model, deep learning technology, applied in neural learning methods, character and pattern recognition, computer parts and other directions, can solve problems such as immaturity
- Summary
- Abstract
- Description
- Claims
- Application Information
AI Technical Summary
Problems solved by technology
Method used
Image
Examples
Embodiment Construction
[0047] Such as figure 2 As shown, the present invention is based on a brain-computer interface-assisted rehabilitation medical co-space model and deep learning method, which combines and improves the two algorithms of CSP and CNN, and performs secondary feature extraction and classification on motor imagery EEG signals. Compared with directly inputting original EEG signals, it not only increases the discrimination between signals, but also reduces the input sample data of CNN from two dimensions to one dimension, greatly reducing the size of input sample data and reducing the number of convolution kernels in the network. The number of network weights that need to be trained is greatly reduced.
[0048] Such as figure 1 As shown, a co-space model and deep learning method based on brain-computer interface assisted rehabilitation medicine, including the following steps:
[0049] Step 1: EEG signal preprocessing, including two processes of wavelet packet transformation and fast...
PUM
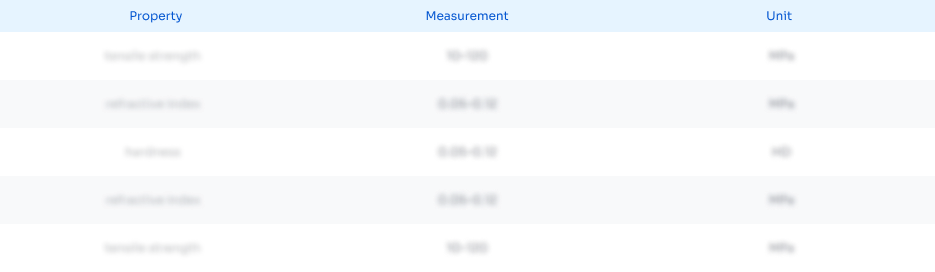
Abstract
Description
Claims
Application Information
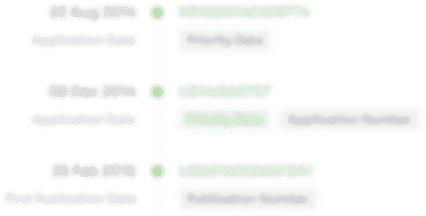
- R&D Engineer
- R&D Manager
- IP Professional
- Industry Leading Data Capabilities
- Powerful AI technology
- Patent DNA Extraction
Browse by: Latest US Patents, China's latest patents, Technical Efficacy Thesaurus, Application Domain, Technology Topic, Popular Technical Reports.
© 2024 PatSnap. All rights reserved.Legal|Privacy policy|Modern Slavery Act Transparency Statement|Sitemap|About US| Contact US: help@patsnap.com