Low resource domain word splitter training method based on transfer learning and word splitting method
A technology of transfer learning and training methods, applied in the field of natural language processing, can solve problems such as a large amount of labeled data, limited number of labeled data, and difficulty in achieving results, so as to achieve smooth learning, improved effects, and reduced conflicts
- Summary
- Abstract
- Description
- Claims
- Application Information
AI Technical Summary
Problems solved by technology
Method used
Image
Examples
Embodiment Construction
[0047] Below in conjunction with accompanying drawing, further describe the present invention through embodiment, but do not limit the scope of the present invention in any way.
[0048] The present invention provides a migration learning method for solving the problem of insufficient resources in word segmentation. By establishing a stacked neural network on the basis of different domain models, the word segmentation model is implemented using data from other resource-rich fields and data from a small amount of domain-specific resources. Training, thereby reducing conflicts in labeling and other aspects between different fields, and learning multi-field data more smoothly, thereby improving the effect of word segmentation in areas with insufficient resources. figure 1 It is a flow chart of the social network text word segmentation method provided by the present invention. The specific process is as follows:
[0049] 1) The input of the algorithm is to segment and mark corpus...
PUM
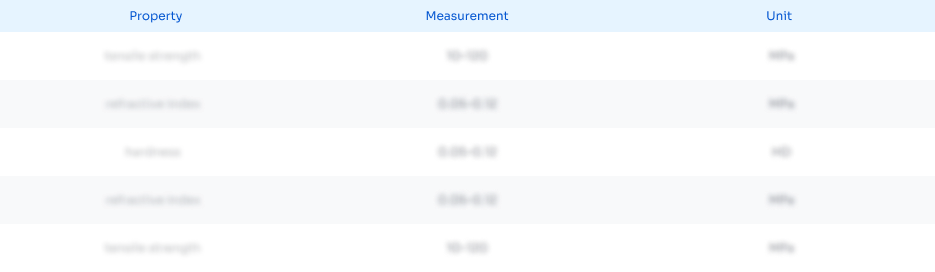
Abstract
Description
Claims
Application Information
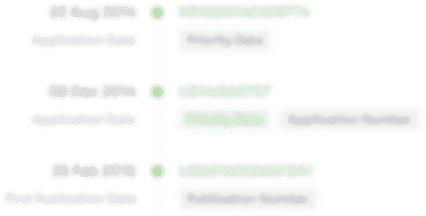
- R&D
- Intellectual Property
- Life Sciences
- Materials
- Tech Scout
- Unparalleled Data Quality
- Higher Quality Content
- 60% Fewer Hallucinations
Browse by: Latest US Patents, China's latest patents, Technical Efficacy Thesaurus, Application Domain, Technology Topic, Popular Technical Reports.
© 2025 PatSnap. All rights reserved.Legal|Privacy policy|Modern Slavery Act Transparency Statement|Sitemap|About US| Contact US: help@patsnap.com