A Bearing Fault Diagnosis Method for Automatic Encoding Machine with Adaptive Parameter Adjustment
An auto-encoding machine and self-adaptive parameter technology, which is applied in self-adaptive control, control/adjustment system, testing of mechanical components, etc., can solve problems such as lack of effect, achieve fast and stable convergence, strong generalization ability, The effect of accurate classification results
- Summary
- Abstract
- Description
- Claims
- Application Information
AI Technical Summary
Problems solved by technology
Method used
Image
Examples
Embodiment Construction
[0047] One, theoretical basis of the present invention.
[0048] 1) The proposal of sparse automatic encoding machine:
[0049] The sparse autoencoder is based on the prototype autoencoder, which imposes constraints on the hidden layer and increases the number of hidden layers accordingly. The sparse encoder can also be compared when the number of neurons in the hidden layer is large. Good for discovering deep features of the input data. The multi-hidden layer sparse autoencoder can extract the sparse explanatory factors of high-dimensional data to retain the non-zero characteristics of the original input. It has relatively good robustness, makes the classification boundary clearer, and can control the scale and change of variables to a certain extent The structure of the input data enhances the presentation ability, comprehensiveness and accuracy of the information. But relatively, if the distribution density of the original data is uneven, the sparse variables obtained aft...
PUM
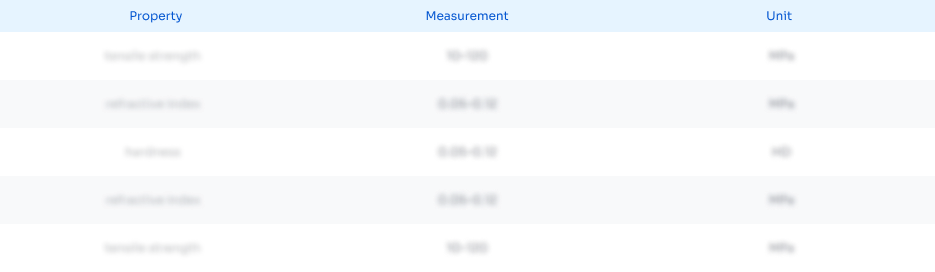
Abstract
Description
Claims
Application Information
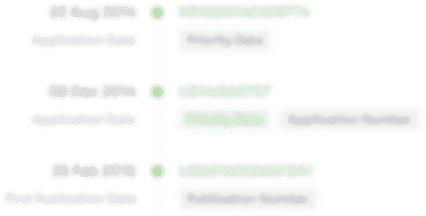
- R&D Engineer
- R&D Manager
- IP Professional
- Industry Leading Data Capabilities
- Powerful AI technology
- Patent DNA Extraction
Browse by: Latest US Patents, China's latest patents, Technical Efficacy Thesaurus, Application Domain, Technology Topic, Popular Technical Reports.
© 2024 PatSnap. All rights reserved.Legal|Privacy policy|Modern Slavery Act Transparency Statement|Sitemap|About US| Contact US: help@patsnap.com