Target classifying method based on local and depth feature assembling
A deep feature and target classification technology, applied to computer parts, character and pattern recognition, instruments, etc., can solve the problems of high computing cost and poor classification effect
- Summary
- Abstract
- Description
- Claims
- Application Information
AI Technical Summary
Problems solved by technology
Method used
Image
Examples
Embodiment Construction
[0036] It should be noted that, in the case of no conflict, the embodiments in the present application and the features in the embodiments can be combined with each other. The present invention will be further described in detail below in conjunction with the drawings and specific embodiments.
[0037] figure 1 It is a system flowchart of an object classification method based on local and deep feature sets of the present invention. It mainly includes deep convolution feature extraction, local feature and encoding and classifier set.
[0038] Deep Convolutional Feature Extraction, Deep Convolutional Features evaluates three popular CNN architectures: AlexNet, VGGNet, and GoogleNet.
[0039] AlexNet's architecture consists of 5 convolutional layers and 3 fully connected layers; it introduces Rectified Linear Units (ReLU) as the use of non-linearities in the pool and ignores neurons during training, thereby reducing overfitting; pooling layers Placed after the first, second and...
PUM
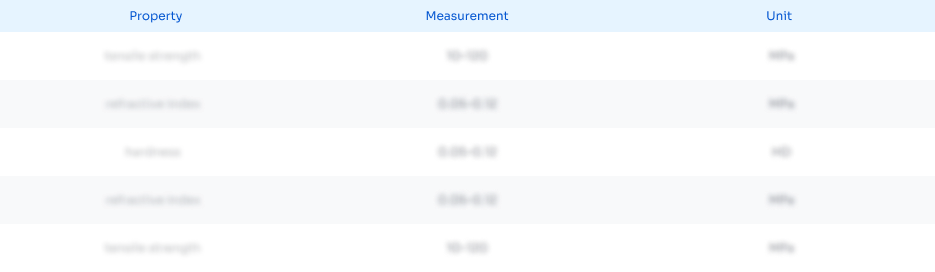
Abstract
Description
Claims
Application Information
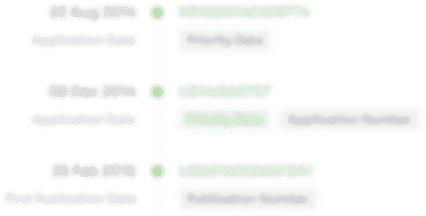
- R&D
- Intellectual Property
- Life Sciences
- Materials
- Tech Scout
- Unparalleled Data Quality
- Higher Quality Content
- 60% Fewer Hallucinations
Browse by: Latest US Patents, China's latest patents, Technical Efficacy Thesaurus, Application Domain, Technology Topic, Popular Technical Reports.
© 2025 PatSnap. All rights reserved.Legal|Privacy policy|Modern Slavery Act Transparency Statement|Sitemap|About US| Contact US: help@patsnap.com