Method for learning non-player character combat strategies on basis of deep Q-learning networks
A non-player character, learning network technology, applied in the field of non-player character combat strategy learning, can solve problems such as low efficiency, poor flexibility, and the inability of players to respond differently, and achieve the effect of reducing labor and rapid automatic adjustment
- Summary
- Abstract
- Description
- Claims
- Application Information
AI Technical Summary
Problems solved by technology
Method used
Image
Examples
Embodiment Construction
[0043] The present invention will be described in further detail below in conjunction with the accompanying drawings and specific embodiments.
[0044] Step (1): Determine the input state set S of the learning NPC; the combat strategy of the learning NPC means that when the learning NPC fights one-on-one with the sparring character, the learning NPC The ability to make different output actions; the characters in the game can be divided into two categories: Learning non-player character (LNPC) and sparring character (Sparring character, SC); the learning non-player character refers to It is a non-player character based on a deep Q-learning network. This type can generate different samples through multiple interactions with sparring characters, so as to continuously learn new combat strategies; sparring characters can be divided into player characters (Playercharacter, PC ) and fixed non-player character (Fixed non-player character, FNPC); the player character refers to the char...
PUM
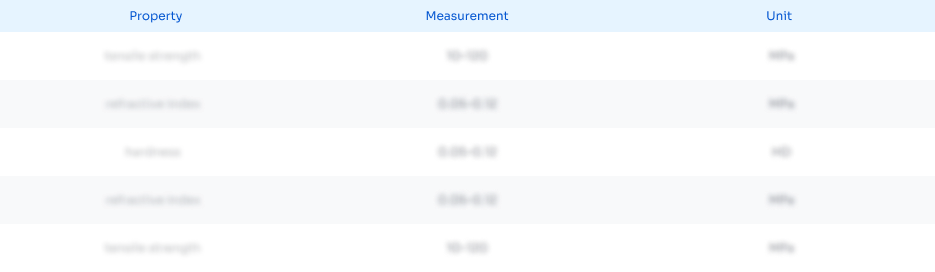
Abstract
Description
Claims
Application Information
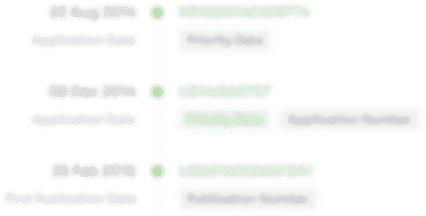
- R&D Engineer
- R&D Manager
- IP Professional
- Industry Leading Data Capabilities
- Powerful AI technology
- Patent DNA Extraction
Browse by: Latest US Patents, China's latest patents, Technical Efficacy Thesaurus, Application Domain, Technology Topic, Popular Technical Reports.
© 2024 PatSnap. All rights reserved.Legal|Privacy policy|Modern Slavery Act Transparency Statement|Sitemap|About US| Contact US: help@patsnap.com