Graded image retrieval method based on deep features of convolutional neural network
A convolutional neural network and deep feature technology, applied in the field of hierarchical image retrieval, can solve the problems that feature compression algorithms are not suitable for two-dimensional features, image representation is not deep, and insufficient utilization, etc., to avoid compression coding operations and decentralized system calculations The effect of increasing the amount and enhancing the retrieval accuracy
- Summary
- Abstract
- Description
- Claims
- Application Information
AI Technical Summary
Problems solved by technology
Method used
Image
Examples
Embodiment Construction
[0037] figure 1 It is the overall flowchart of the image retrieval system of the present invention. The image retrieval process is divided into four steps:
[0038] The first step is to set the parameters of the feature extraction network:
[0039] The VGG network architecture with deep layers in CNN is used as the feature extraction network.
[0040] figure 2 It is a schematic diagram of the network structure of the VGG network.
[0041] The VGG network adopts a multi-hidden layer structure to classify the input image, and a three-channel image with a size of 224*224 is input into the network through an input layer, and image features are extracted through five convolution modules and a fully connected layer module, and finally used These features output probabilities for all classes at the output layer. Among them, the first four convolution modules adopt the structure of single convolution layer and ReLU layer, and the fifth convolution module includes the structure o...
PUM
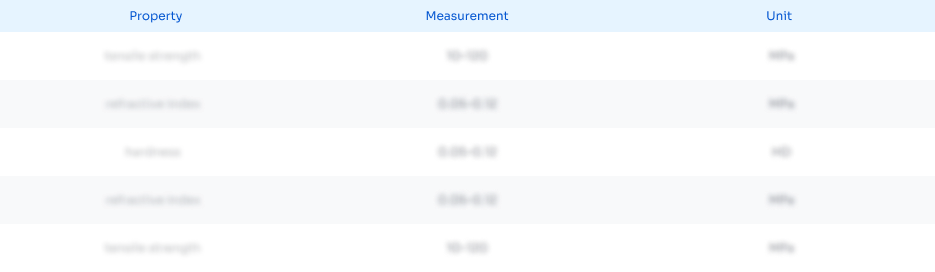
Abstract
Description
Claims
Application Information
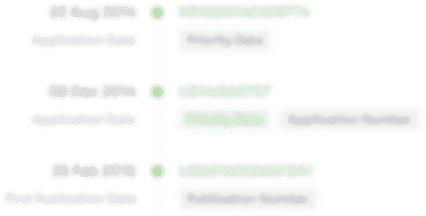
- R&D
- Intellectual Property
- Life Sciences
- Materials
- Tech Scout
- Unparalleled Data Quality
- Higher Quality Content
- 60% Fewer Hallucinations
Browse by: Latest US Patents, China's latest patents, Technical Efficacy Thesaurus, Application Domain, Technology Topic, Popular Technical Reports.
© 2025 PatSnap. All rights reserved.Legal|Privacy policy|Modern Slavery Act Transparency Statement|Sitemap|About US| Contact US: help@patsnap.com