A deep learning network training method for video satellite super-resolution reconstruction
A deep learning network and super-resolution reconstruction technology, applied in the field of machine learning, can solve problems such as limitations, not considering the relative influence of pixel grayscale, and achieve the effect of promoting performance improvement and improving training effect.
- Summary
- Abstract
- Description
- Claims
- Application Information
AI Technical Summary
Problems solved by technology
Method used
Image
Examples
Embodiment Construction
[0015] In order to facilitate those of ordinary skill in the art to understand and implement the present invention, the present invention will be described in further detail below in conjunction with the examples. It should be understood that the implementation examples described here are only used to illustrate and explain the present invention, and are not intended to limit the present invention.
[0016] The dynamic video of the video satellite has inherent insufficient spatial resolution and blurring limitations, and using its own image as a training sample cannot provide sufficient high-frequency information, which seriously restricts the degree of detail restoration of the reconstructed high-resolution image. Compared with dynamic satellite video, under the same sensor sampling and channel transmission throughput conditions, the spatial resolution of static satellite imagery is much higher and the details of ground objects are more abundant. Therefore, static satellite im...
PUM
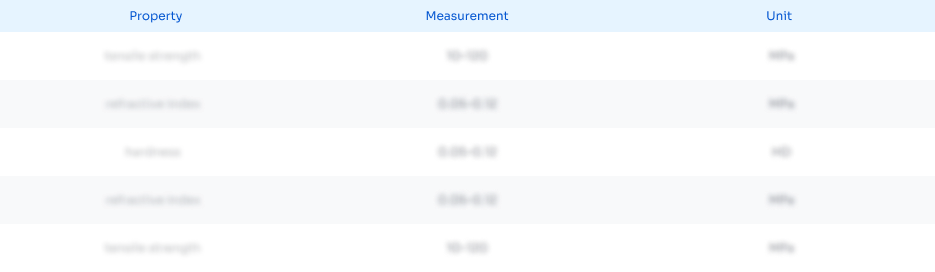
Abstract
Description
Claims
Application Information
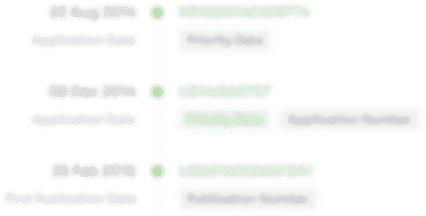
- R&D
- Intellectual Property
- Life Sciences
- Materials
- Tech Scout
- Unparalleled Data Quality
- Higher Quality Content
- 60% Fewer Hallucinations
Browse by: Latest US Patents, China's latest patents, Technical Efficacy Thesaurus, Application Domain, Technology Topic, Popular Technical Reports.
© 2025 PatSnap. All rights reserved.Legal|Privacy policy|Modern Slavery Act Transparency Statement|Sitemap|About US| Contact US: help@patsnap.com