Class-imbalance problem classification method based on expansion training data set
A training data set, problem classification technology, applied in character and pattern recognition, instruments, computer parts and other directions, can solve problems such as limited improvement in accuracy, relatively limited improvement, and low time complexity, and achieve improved results and good results. effect, the effect of improving the classification accuracy
- Summary
- Abstract
- Description
- Claims
- Application Information
AI Technical Summary
Problems solved by technology
Method used
Image
Examples
Embodiment
[0033] The problem of class imbalance is a common problem in the process of obtaining data sets. It is specifically manifested as: the number of samples of a certain class in the data set is far from the number of other samples. For example, in the data set of credit card fraud, the behavior of most users is normal, and only a very small number of users will be judged as fraudulent. If the data set or algorithm is not improved accordingly, and the classification training is carried out directly, the result is that the sample data of the minority class will not be given sufficient attention, and in severe cases, it will even be ignored by the classifier as noise, resulting in poor classification results. Serious deviation.
[0034] In this context, how to obtain our ideal results from category-imbalanced data has become a problem that requires in-depth exploration. At present, there are two main types of optimization methods for the imbalance problem: (1) change the original d...
PUM
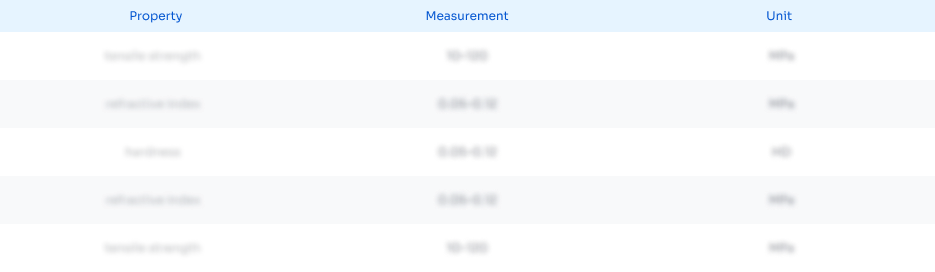
Abstract
Description
Claims
Application Information
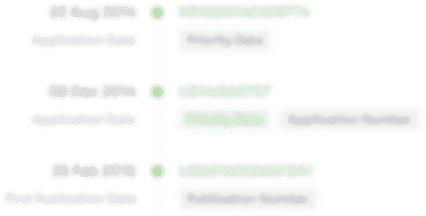
- R&D Engineer
- R&D Manager
- IP Professional
- Industry Leading Data Capabilities
- Powerful AI technology
- Patent DNA Extraction
Browse by: Latest US Patents, China's latest patents, Technical Efficacy Thesaurus, Application Domain, Technology Topic, Popular Technical Reports.
© 2024 PatSnap. All rights reserved.Legal|Privacy policy|Modern Slavery Act Transparency Statement|Sitemap|About US| Contact US: help@patsnap.com