Auto-encoder and clustering-based hybrid recommendation method
A technology of mixed recommendation and recommendation method, applied in the direction of neural learning method, computer parts, character and pattern recognition, etc., can solve problems such as not considered, and achieve the effect of improving accuracy
- Summary
- Abstract
- Description
- Claims
- Application Information
AI Technical Summary
Problems solved by technology
Method used
Image
Examples
Embodiment Construction
[0062] The present invention will be further explained below in conjunction with the accompanying drawings and specific embodiments.
[0063] like Figure 1-5 Show, the present invention comprises the steps:
[0064] (1) Combining user rating matrix with user demographic characteristics;
[0065] (2) Use an autoencoder to learn user features, and use the obtained user features to cluster users;
[0066] (3) Use MAE to calculate the most suitable recommendation method for each category of users, and combine the recommendation methods to obtain a hybrid recommendation model;
[0067] (4) Calculate the target user category, and use the hybrid recommendation model to get the recommendation result.
[0068] In step (1), the specific steps of combining the user rating matrix and user demographic characteristics are as follows:
[0069] (1.1) Let the user data set U={U1, U2,...,Un}, the project data set I={I1,I2,...,Im}, and the user's rating range for the project is [0,5];
[0...
PUM
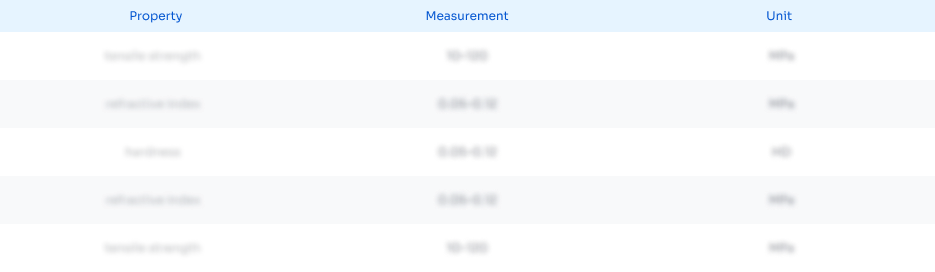
Abstract
Description
Claims
Application Information
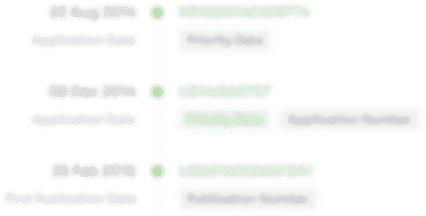
- R&D
- Intellectual Property
- Life Sciences
- Materials
- Tech Scout
- Unparalleled Data Quality
- Higher Quality Content
- 60% Fewer Hallucinations
Browse by: Latest US Patents, China's latest patents, Technical Efficacy Thesaurus, Application Domain, Technology Topic, Popular Technical Reports.
© 2025 PatSnap. All rights reserved.Legal|Privacy policy|Modern Slavery Act Transparency Statement|Sitemap|About US| Contact US: help@patsnap.com