Extraction method of entity relationship of electronic medical record, based on BLSTM and attention mechanism
A technology of entity relationship and electronic medical records, applied in neural learning methods, electrical digital data processing, special data processing applications, etc., can solve problems such as dependence
- Summary
- Abstract
- Description
- Claims
- Application Information
AI Technical Summary
Problems solved by technology
Method used
Image
Examples
Embodiment Construction
[0011] Below in conjunction with specific embodiment the present invention is described in further detail:
[0012] 1. Get the input basic feature vector representation
[0013] The basic feature vector is mainly composed of three parts of the input sentence itself (W), the relative distance between each word and the entity pair, and the word type.
[0014] 1) Features of the word itself (W):
[0015] For a given sentence S={x with n words 1 , x 2 ,...,x n}, we first convert each word into a low-dimensional real vector using the word2vec toolkit. The word representation is through the embedding matrix Encoded by column vectors in , where V is a fixed-size dictionary, d w is the size of the embedding matrix
[0016] 2) The relative distance feature of each word to entity pair:
[0017] we use Matrix to represent the distance of each word to entity pair, where d d is the dimension after each relative distance is mapped to a real vector, and is a hyperparameter that c...
PUM
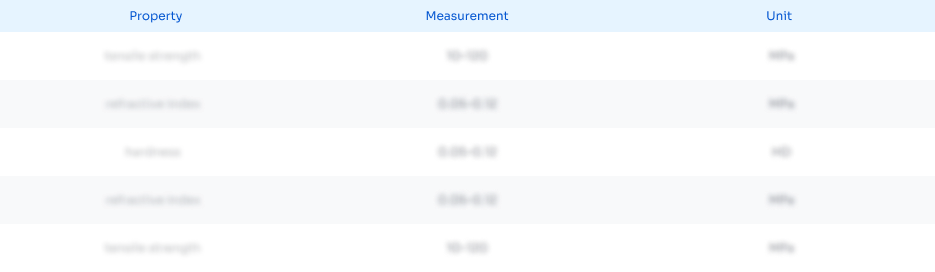
Abstract
Description
Claims
Application Information
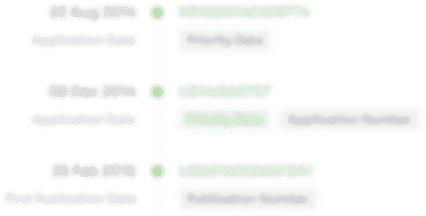
- R&D Engineer
- R&D Manager
- IP Professional
- Industry Leading Data Capabilities
- Powerful AI technology
- Patent DNA Extraction
Browse by: Latest US Patents, China's latest patents, Technical Efficacy Thesaurus, Application Domain, Technology Topic, Popular Technical Reports.
© 2024 PatSnap. All rights reserved.Legal|Privacy policy|Modern Slavery Act Transparency Statement|Sitemap|About US| Contact US: help@patsnap.com