Least Squares Support Vector Machine Power Forecasting Method Based on Maximum Correlation Entropy Criterion
A support vector machine and maximum correlation entropy technology, applied in forecasting, data processing applications, instruments, etc., can solve the problems of low accuracy of electricity sales forecast model and difficulty in meeting the requirements of electricity sales transactions, etc., to achieve fast calculation speed and data requirements The effect of small amount and local similarity improvement
- Summary
- Abstract
- Description
- Claims
- Application Information
AI Technical Summary
Problems solved by technology
Method used
Image
Examples
Embodiment
[0057] Step 1. Use the electricity consumption of a machinery manufacturing enterprise in a certain city for the same period of three years and the corresponding monthly average temperature to establish an input data set.
[0058] Step 2. For the concentrated and missing data of historical electricity consumption data, the electricity consumption in the same period of the previous year and the electricity consumption in the previous and next months of the same year are added and averaged to supplement.
[0059] Step 3. Normalize the input data set.
[0060] Step 4. Apply the following formula to predict the power
[0061]
[0062] where x trian is the input of the training set, x test As the input of the test set, bring it into the formula to get the final prediction result y i .
[0063] Step 5. Introduce K-fold cross-validation and grid optimization method to the key parameter σ of the model 0 Optimize with σ, and use the maximum correlation entropy criterion instead...
PUM
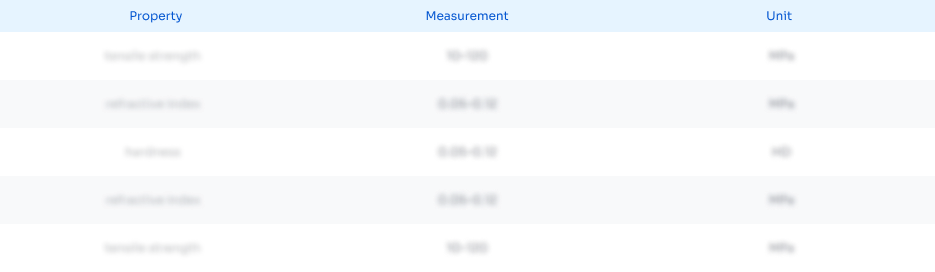
Abstract
Description
Claims
Application Information
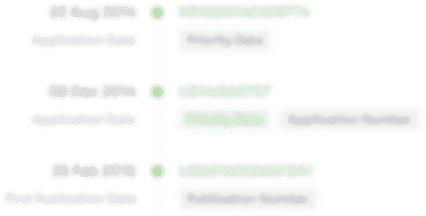
- R&D
- Intellectual Property
- Life Sciences
- Materials
- Tech Scout
- Unparalleled Data Quality
- Higher Quality Content
- 60% Fewer Hallucinations
Browse by: Latest US Patents, China's latest patents, Technical Efficacy Thesaurus, Application Domain, Technology Topic, Popular Technical Reports.
© 2025 PatSnap. All rights reserved.Legal|Privacy policy|Modern Slavery Act Transparency Statement|Sitemap|About US| Contact US: help@patsnap.com