A method and system for fruit recognition and tracking based on deep learning algorithm
A deep learning and fruit recognition technology, applied in the field of recognition and tracking, can solve the problems of low target recognition accuracy, easy loss of targets, and poor target positioning accuracy
- Summary
- Abstract
- Description
- Claims
- Application Information
AI Technical Summary
Problems solved by technology
Method used
Image
Examples
Embodiment 1
[0051] Embodiment 1 of the present invention provides a method for identifying and tracking fruits based on deep learning algorithms, such as figure 1 As shown, the method includes:
[0052] S1: Acquire the image of the pre-tracking part;
[0053] S2: Use the deep learning algorithm to identify the target in the image, and obtain the position of the target in the image;
[0054] S3: Track the location of the target.
[0055] needs to be specified, such as figure 2 As shown, step S2 specifically includes:
[0056] S21: Use the deep recognition network algorithm to identify target features, and calculate the confidence of each target feature;
[0057] S22: Compare the confidence of each target feature with a confidence threshold, and when there is a target feature greater than the confidence threshold, proceed to step S23;
[0058] S23: Determine the number N corresponding to the target feature greater than the confidence threshold, if N=1, go to step S24, if N>1, go to st...
Embodiment 2
[0067] Embodiment 2 of the present invention provides a fruit recognition and tracking method based on a deep learning algorithm, which is basically the same as that of Embodiment 1, the difference is that the preset number of frames is 100 frames, and by setting the preset number of frames to 100 frame, which can ensure the timely recognition and tracking of the next frame of images, and ensure the efficiency of recognition and tracking. If the number of frames is too small, there will be recognition and tracking process interleaving, which will cause errors in recognition and tracking. If the number of frames is too large, there will be recognition and tracking errors. Tracking is discontinuous, reducing work efficiency.
Embodiment 3
[0069] Embodiment 3 of the present invention provides a method for identifying and tracking fruits based on a deep learning algorithm, which is basically the same as that in Embodiment 2. The difference is that, as Figure 4 As shown, step S3 also includes:
[0070] S34: When step S32 judges that the target is not lost, judge the time t tracked by the depth tracking network algorithm and compare it with the time threshold t 1 Make a judgment, when t1 , proceed to step S35, when t≥t 1 , proceed to step S2;
[0071] S35: sending a picking instruction to the robot;
[0072] S36: When step S32 determines that the target is lost, at the same time send an instruction to the robot to stop moving until step S35 is performed.
[0073] In the identification tracking method provided by the present invention, the tracking time is further judged, and when the tracking time reaches a preset threshold, step S2 is performed again. This improves the overall tracking efficiency; when the ta...
PUM
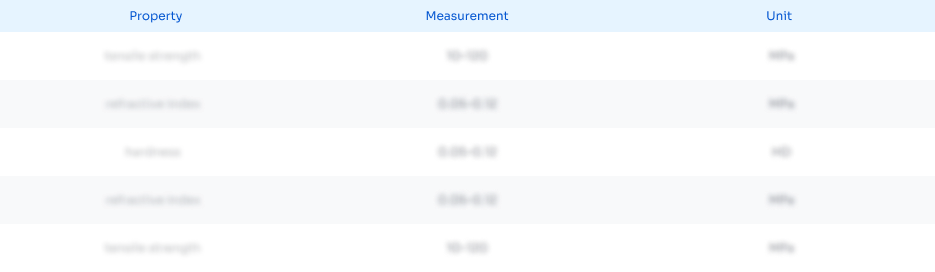
Abstract
Description
Claims
Application Information
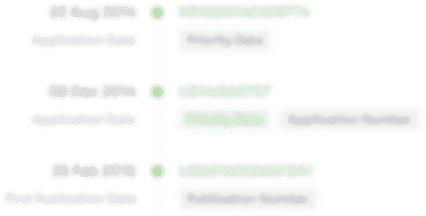
- R&D
- Intellectual Property
- Life Sciences
- Materials
- Tech Scout
- Unparalleled Data Quality
- Higher Quality Content
- 60% Fewer Hallucinations
Browse by: Latest US Patents, China's latest patents, Technical Efficacy Thesaurus, Application Domain, Technology Topic, Popular Technical Reports.
© 2025 PatSnap. All rights reserved.Legal|Privacy policy|Modern Slavery Act Transparency Statement|Sitemap|About US| Contact US: help@patsnap.com