Data dimensionality reduction method for two-dimensional time-frequency data
A data dimensionality reduction, time-frequency technology, used in instruments, character and pattern recognition, computer parts, etc., can solve the problems of unable to use the nonlinear characteristics of data, and the recognition rate cannot reach the ideal.
- Summary
- Abstract
- Description
- Claims
- Application Information
AI Technical Summary
Problems solved by technology
Method used
Image
Examples
Embodiment Construction
[0030] In the following, the algorithm of the present invention is used to reduce the dimensionality of the wavelet transform two-dimensional time-frequency representation data of the radar high-resolution range image, and obtain its projection transformation matrix. This example is used to describe the implementation of the present invention in detail, so as to have a deeper understanding of how to apply the technical means of the present invention to solve technical problems, in order to achieve the purpose of solving practical problems well, and implement accordingly. The present invention kernel two-dimensional two-dimensional principal component analysis algorithm, the implementation steps of the present invention are as follows figure 1 As shown, each step is specifically implemented in the following manner:
[0031] Step 1: Centralize each range image time-frequency representation sample A i ∈R m × R n (i=1,2,...,M, M is the number of time-frequency sample matrix): ...
PUM
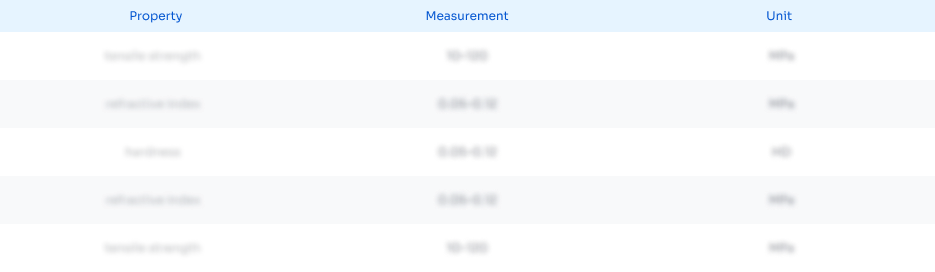
Abstract
Description
Claims
Application Information
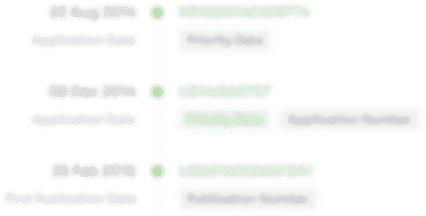
- R&D Engineer
- R&D Manager
- IP Professional
- Industry Leading Data Capabilities
- Powerful AI technology
- Patent DNA Extraction
Browse by: Latest US Patents, China's latest patents, Technical Efficacy Thesaurus, Application Domain, Technology Topic, Popular Technical Reports.
© 2024 PatSnap. All rights reserved.Legal|Privacy policy|Modern Slavery Act Transparency Statement|Sitemap|About US| Contact US: help@patsnap.com