A Convolutional Neural Network Based Defect Identification Method for Solar Panels
A solar panel and convolutional neural network technology, applied in the field of solar panel defect identification based on convolutional neural network, can solve problems such as inability to perform effective detection, and achieve the effect of wide applicability
- Summary
- Abstract
- Description
- Claims
- Application Information
AI Technical Summary
Problems solved by technology
Method used
Image
Examples
Embodiment 1
[0120] In this embodiment, a method for identifying defects of a solar panel based on a convolutional neural network includes two stages of model offline training and online detection.
[0121] The offline training of the model includes the following steps:
[0122] S1: Collect qualified images and multi-category defect images of solar panels, and complete classification. There are four types of defects: open welding, broken grid, shadow, and hidden cracks. The number of sample pictures obtained are 14, 32, 72, and 10 respectively. In addition, there are 9 unclassified defect sample pictures. The number of qualified sample pictures is 1500.
[0123] S2: Perform data balance on the images of each category obtained in step S1, so that the number of samples in each category is sufficient and has approximately the same number of samples, so as to facilitate model training: for the four types of defect images with a small number, use left-right flip and up-down flip , 180° rotati...
PUM
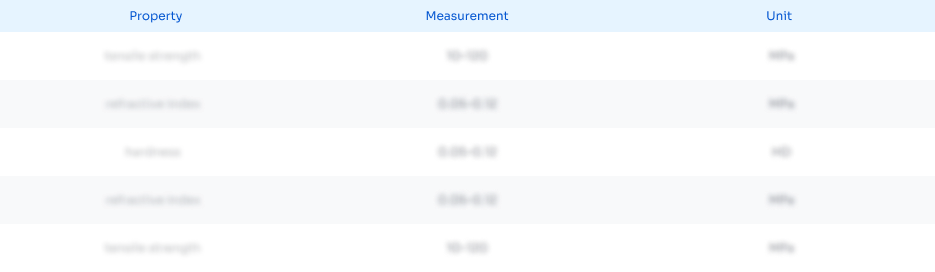
Abstract
Description
Claims
Application Information
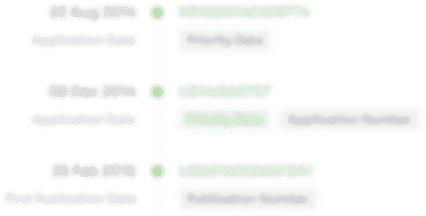
- R&D Engineer
- R&D Manager
- IP Professional
- Industry Leading Data Capabilities
- Powerful AI technology
- Patent DNA Extraction
Browse by: Latest US Patents, China's latest patents, Technical Efficacy Thesaurus, Application Domain, Technology Topic, Popular Technical Reports.
© 2024 PatSnap. All rights reserved.Legal|Privacy policy|Modern Slavery Act Transparency Statement|Sitemap|About US| Contact US: help@patsnap.com