A data preprocessing method for ECG signal classification based on deep learning model
An electrocardiographic signal and deep learning technology, applied in signal pattern recognition, character and pattern recognition, instruments, etc., can solve the problems of sample imbalance, deep learning model difficult to train, and the total sample size is scarce, and achieve sample balance. , easy to train, increase the effect of training samples
- Summary
- Abstract
- Description
- Claims
- Application Information
AI Technical Summary
Problems solved by technology
Method used
Image
Examples
Embodiment Construction
[0031] The present invention will be further described below in conjunction with the accompanying drawings.
[0032] refer to Figure 1 ~ Figure 4 , a data preprocessing method for ECG signal classification based on a deep learning model, comprising the following steps:
[0033] a. Obtain ECG signals marked by experts including normal ECG and abnormal ECG as training samples. The measurement time of ECG signals is arbitrary. Assume that the longest measurement time is t seconds, the sampling frequency is fs Hz, and there are L abnormal ECG signal types. category, the number of samples of normal ECG signals is N, the number of samples of category 1 abnormal ECG signals is N1, the number of samples of category 2 abnormal ECG signals is N2...the number of samples of category L abnormal ECG signals is NL;
[0034] b Perform noise reduction processing on the original training samples, and use wavelet transform to remove baseline drift;
[0035] c Divide the training sample into a...
PUM
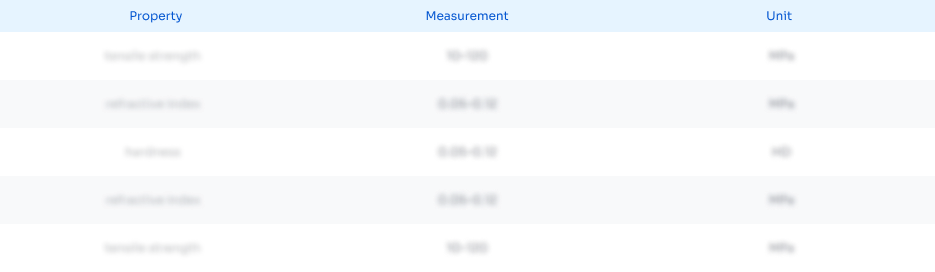
Abstract
Description
Claims
Application Information
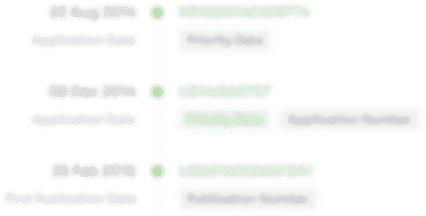
- R&D Engineer
- R&D Manager
- IP Professional
- Industry Leading Data Capabilities
- Powerful AI technology
- Patent DNA Extraction
Browse by: Latest US Patents, China's latest patents, Technical Efficacy Thesaurus, Application Domain, Technology Topic, Popular Technical Reports.
© 2024 PatSnap. All rights reserved.Legal|Privacy policy|Modern Slavery Act Transparency Statement|Sitemap|About US| Contact US: help@patsnap.com