Random forest integration method improved through width neural network
A technology of random forest and integration method, applied in the field of random forest integration improved by wide neural network, can solve the problems of deep forest parallelization limitation, lack of rationality, etc., achieve high degree of automation, improve training efficiency, and strong parallelization ability Effect
- Summary
- Abstract
- Description
- Claims
- Application Information
AI Technical Summary
Problems solved by technology
Method used
Image
Examples
Embodiment Construction
[0031] Specific embodiments of the present invention will be further described below in conjunction with accompanying drawings
[0032] A random forest integration method based on feature mapping layer and enhancement layer structure, characterized in that: it includes two parts of model design and model training;
[0033] For samples with a spatial position relationship between image or input data features, this method is used to generate the feature input vector of the feature mapping layer. For the input without spatial position relationship, the original input is directly used as the input feature vector of the feature mapping layer.
[0034] Such as figure 1 The overall flow chart of the model is shown, in which the input layer performs multi-granularity scanning on the data with spatial position relationship to obtain the input feature vector. However, for the original input that is not associated with the spatial position, it can be directly used as the input feature v...
PUM
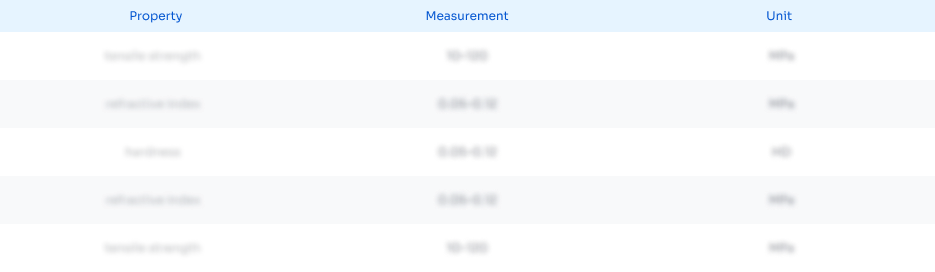
Abstract
Description
Claims
Application Information
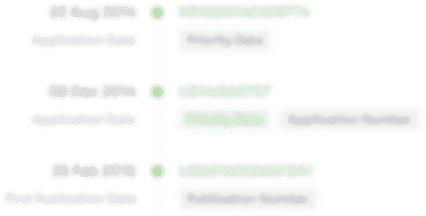
- R&D Engineer
- R&D Manager
- IP Professional
- Industry Leading Data Capabilities
- Powerful AI technology
- Patent DNA Extraction
Browse by: Latest US Patents, China's latest patents, Technical Efficacy Thesaurus, Application Domain, Technology Topic.
© 2024 PatSnap. All rights reserved.Legal|Privacy policy|Modern Slavery Act Transparency Statement|Sitemap