Semantic segmentation method based on improved full convolutional neural network
A convolutional neural network and semantic segmentation technology, applied in the field of computer vision, can solve the problems of loss of image detail sensitivity and feature resolution reduction, and achieve the effect of enriching feature map details, expanding receptive field, and improving accuracy
- Summary
- Abstract
- Description
- Claims
- Application Information
AI Technical Summary
Problems solved by technology
Method used
Image
Examples
Embodiment Construction
[0020] The specific implementation of the present invention will be further described in detail below in conjunction with the accompanying drawings of the embodiments, so as to make the technical solution of the present invention easier to understand and grasp, so as to define and support the protection scope of the present invention more clearly.
[0021] Such as figure 1 As shown, the present invention designs a semantic segmentation method for improving the full convolutional network, and obtains a multi-hole full convolutional network based on the improvement of the full convolutional neural network, and performs end-to-end training on it. This method specifically comprises the following steps:
[0022] Step 1. Obtain training image data.
[0023] Since the network layer is relatively deep and the number of parameters required for training is large, the amount of training data that needs to be prepared needs to meet a certain order of magnitude requirement. The PASCAL VO...
PUM
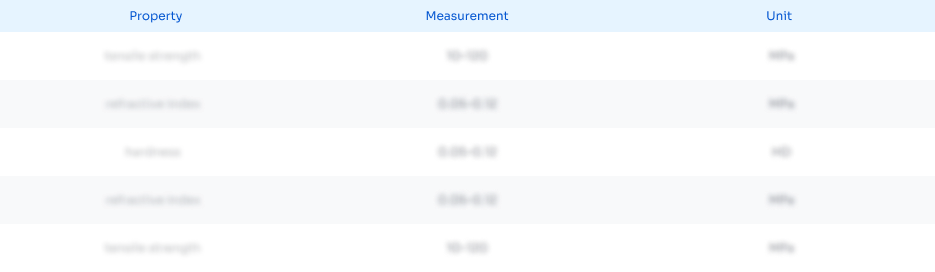
Abstract
Description
Claims
Application Information
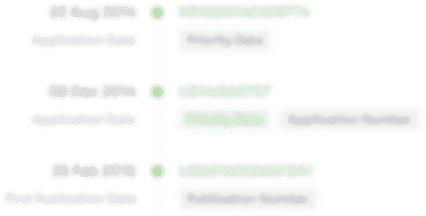
- R&D Engineer
- R&D Manager
- IP Professional
- Industry Leading Data Capabilities
- Powerful AI technology
- Patent DNA Extraction
Browse by: Latest US Patents, China's latest patents, Technical Efficacy Thesaurus, Application Domain, Technology Topic, Popular Technical Reports.
© 2024 PatSnap. All rights reserved.Legal|Privacy policy|Modern Slavery Act Transparency Statement|Sitemap|About US| Contact US: help@patsnap.com