Image classification method based on direct push semi-supervised depth learning
A technology of deep learning and classification methods, applied in neural learning methods, character and pattern recognition, instruments, etc., can solve problems such as errors, affecting the stability and effect of model training, misleading model training, etc.
- Summary
- Abstract
- Description
- Claims
- Application Information
AI Technical Summary
Problems solved by technology
Method used
Image
Examples
Embodiment Construction
[0066] In view of the above research status, the present invention proposes a transductive semi-supervised deep learning (TSSDL) algorithm capable of effectively training a deep convolutional neural network model. The proposed Transductive Semi-Supervised Deep Learning (TSSDL) algorithm mainly consists of three parts: First, the present invention extends the traditional TSSL method to make it suitable for DCNN training. The present invention takes the labels of unlabeled samples as variables, minimizes the loss function through iterative training, and simultaneously determines the optimal unlabeled sample labels and deep convolutional neural network model parameters. As far as the present invention knows, the method proposed by the present invention is the first attempt to apply the conduction learning principle to the deep convolutional neural network model training process. Secondly, in order to overcome the problem that the low-quality feature descriptors generated by the d...
PUM
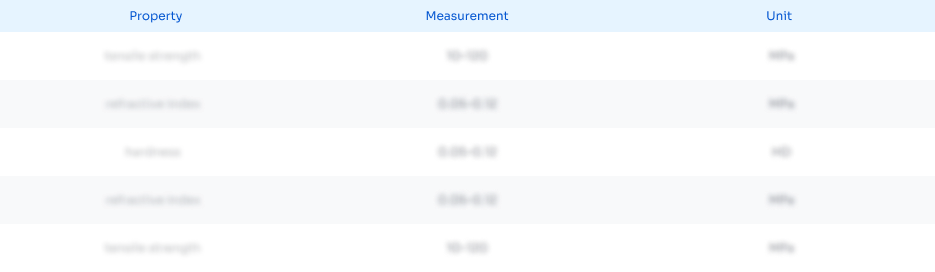
Abstract
Description
Claims
Application Information
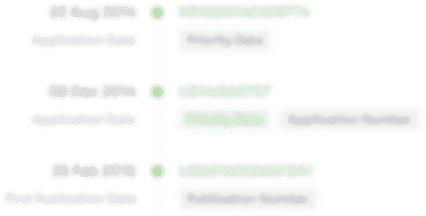
- Generate Ideas
- Intellectual Property
- Life Sciences
- Materials
- Tech Scout
- Unparalleled Data Quality
- Higher Quality Content
- 60% Fewer Hallucinations
Browse by: Latest US Patents, China's latest patents, Technical Efficacy Thesaurus, Application Domain, Technology Topic, Popular Technical Reports.
© 2025 PatSnap. All rights reserved.Legal|Privacy policy|Modern Slavery Act Transparency Statement|Sitemap|About US| Contact US: help@patsnap.com