Batch A3C reinforcement learning method for agent exploring 3D maze
A technology of reinforcement learning and intelligent agents, applied in the direction of neural learning methods, neural architecture, biological neural network models, etc., can solve problems such as inconsistency and RL powerlessness
- Summary
- Abstract
- Description
- Claims
- Application Information
AI Technical Summary
Problems solved by technology
Method used
Image
Examples
Embodiment Construction
[0024] In order to further illustrate the purpose, technical solution and features of the present invention, the present invention will be further described below in conjunction with examples of implementing the method and with reference to the accompanying drawings. The invention adopts the batch-based A3C deep reinforcement learning method to realize the agent to explore the 3D maze. Use CNN-MLP to extract the low-dimensional feature vec of the agent's state, and then use LSTM+MLP to respectively predict the prediction of the agent's action distribution based on the current state, and the state value of the current state. This state value approximates the reconstructed action value function . The improvement of the present invention can be summarized in the following two aspects: 1) only one set of neural network parameters is needed, and the deep reinforcement learning based on the A3C algorithm can be completed with the help of experience pool and process technology to com...
PUM
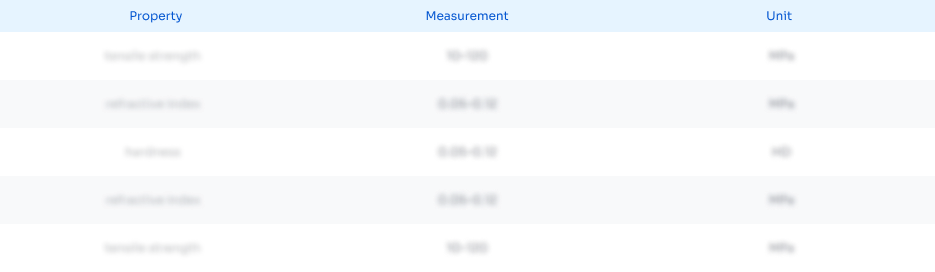
Abstract
Description
Claims
Application Information
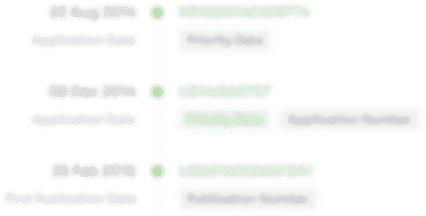
- R&D Engineer
- R&D Manager
- IP Professional
- Industry Leading Data Capabilities
- Powerful AI technology
- Patent DNA Extraction
Browse by: Latest US Patents, China's latest patents, Technical Efficacy Thesaurus, Application Domain, Technology Topic.
© 2024 PatSnap. All rights reserved.Legal|Privacy policy|Modern Slavery Act Transparency Statement|Sitemap