Semantic Image Segmentation Method Using Convolutional Neural Networks
A convolutional neural network and image segmentation technology, which is applied in the direction of instruments, computing, character and pattern recognition, etc., can solve the problems of information loss, failure to make good use of information, and failure to consider pixel connection, etc., to achieve accurate results and Smoothing, accuracy-enhancing effects
- Summary
- Abstract
- Description
- Claims
- Application Information
AI Technical Summary
Problems solved by technology
Method used
Image
Examples
Embodiment Construction
[0022] In order to make the object, technical solution and advantages of the present invention clearer, the present invention will be further described in detail below in conjunction with the accompanying drawings.
[0023] The designer of the present invention has devoted himself to the research of semantic image segmentation, summed up and aimed at the deficiencies and drawbacks of the current existing technology, and innovatively proposed a semantic image segmentation method of convolutional neural network, by combining partial layered feature fusion and Fully connected conditional random field processing for semantic image segmentation. The overview steps include: 1. Construct a network architecture model consisting of convolutional layers, pooling layers, upsampling layers, and loss functions, and the step size, convolution kernel size, and output characteristics of each layer in the model The number of graphs is customized according to the specifications; 2. Fusion of sh...
PUM
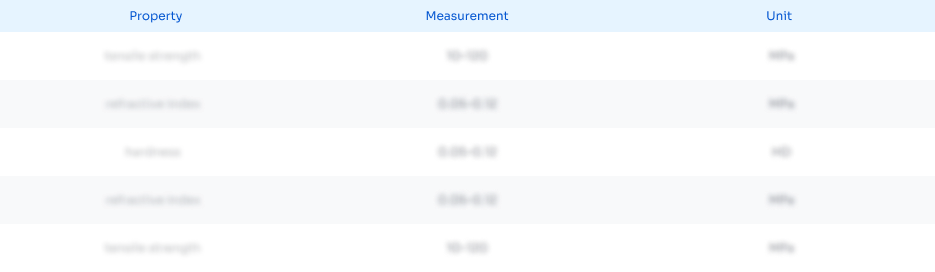
Abstract
Description
Claims
Application Information
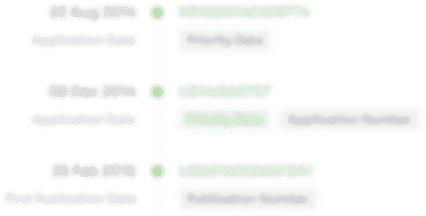
- Generate Ideas
- Intellectual Property
- Life Sciences
- Materials
- Tech Scout
- Unparalleled Data Quality
- Higher Quality Content
- 60% Fewer Hallucinations
Browse by: Latest US Patents, China's latest patents, Technical Efficacy Thesaurus, Application Domain, Technology Topic, Popular Technical Reports.
© 2025 PatSnap. All rights reserved.Legal|Privacy policy|Modern Slavery Act Transparency Statement|Sitemap|About US| Contact US: help@patsnap.com