Deep learning-based ICU death risk evaluation system
A deep learning and evaluation system technology, applied in the field of death risk prediction, can solve problems such as inability to assess the risk of death of patients, rough output results, etc., and achieve the effect of close correlation and improved efficiency
- Summary
- Abstract
- Description
- Claims
- Application Information
AI Technical Summary
Problems solved by technology
Method used
Image
Examples
Embodiment 1
[0034] Please refer to figure 1 As shown, the present invention discloses a deep learning-based ICU death risk assessment system, which includes an ICU historical database, a first data preprocessing module, a death risk assessment module, a second data preprocessing module, and a human-computer interaction module; The ICU historical database, the first data preprocessing module, the death risk assessment module and the human-computer interaction module are connected in sequence, and the human-computer interaction module, the second data preprocessing module and the death risk assessment module are connected in sequence.
[0035] The historical patient's sign data set and the real final state of the historical patient are stored in the ICU historical database. The first data preprocessing module extracts the historical patient's sign data set from the ICU historical database and performs preprocessing to obtain training sample data, and at the same time extracts the real final...
Embodiment 2
[0058] In this embodiment, the death risk assessment modules are respectively constructed using a one-way LSTM architecture and a two-way LSTM architecture, and the schematic diagrams of the models are as follows image 3 and Figure 4 shown, with figure 2 It can be seen from the comparison that for the models of the two LSTM architectures, the results are only output at the last time step, and the loss value Loss is calculated with the label Label to train the model.
[0059] Such as Figure 5 Shown is the evaluation effect comparison table of the ICU mortality risk assessment system constructed and trained using the unidirectional LSTM architecture (LSTM), the bidirectional LSTM architecture (BiLSTM) and the bidirectional supervised LSTM architecture (BiLSTM-ST). The ICU mortality risk assessment model based on the two-way supervised LSTM architecture is significantly better than that based on LSTM or BiLSTM architecture in terms of Precision (precision rate), Recall (rec...
PUM
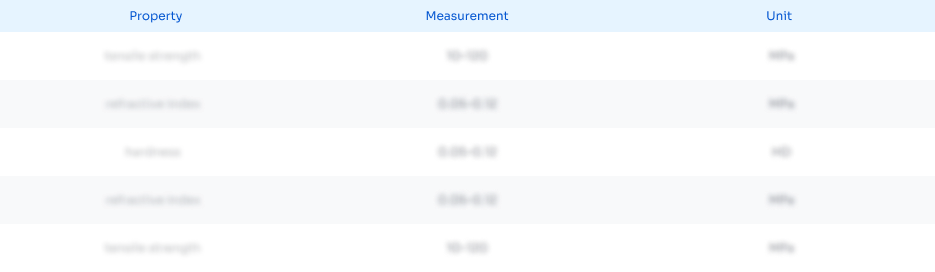
Abstract
Description
Claims
Application Information
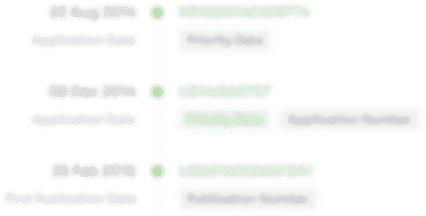
- R&D Engineer
- R&D Manager
- IP Professional
- Industry Leading Data Capabilities
- Powerful AI technology
- Patent DNA Extraction
Browse by: Latest US Patents, China's latest patents, Technical Efficacy Thesaurus, Application Domain, Technology Topic, Popular Technical Reports.
© 2024 PatSnap. All rights reserved.Legal|Privacy policy|Modern Slavery Act Transparency Statement|Sitemap|About US| Contact US: help@patsnap.com