Rotating machinery health assessment method for deep self-encoding network
A self-encoding network and deep self-encoding technology, applied in the field of rotating machinery health assessment of deep self-encoding network, can solve the problem that cannot be used to accurately evaluate rotating machinery health indicators, health indicators monotonicity, trend robustness needs to be improved, etc. question
- Summary
- Abstract
- Description
- Claims
- Application Information
AI Technical Summary
Problems solved by technology
Method used
Image
Examples
Embodiment Construction
[0039] The present invention will be further described in detail below in conjunction with the accompanying drawings and specific preferred embodiments.
[0040] Such as figure 1 As shown, a rotating machinery health assessment method based on a deep autoencoder network includes the following steps.
[0041] Step 1, vibration signal collection: collect the vibration signals of the key parts of the rotating machinery.
[0042] Key components of rotating machinery include bearings, gears or rotors etc.
[0043] The acquisition method of the vibration signal of key components is an existing technology. In this application, taking the bearing as an example, the following optimal method is adopted for acquisition.
[0044] Step 11, bearing installation: install four bearings on the bearing life enhancement test bench at the same time, the model of the bearing life enhancement test bench is preferably ABLT-1A, which includes the test head, test head seat, transmission system, load...
PUM
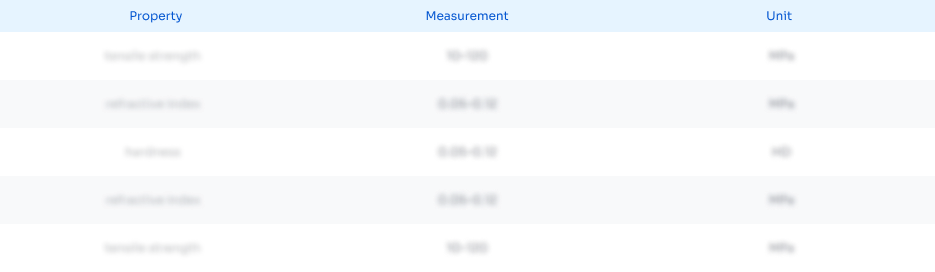
Abstract
Description
Claims
Application Information
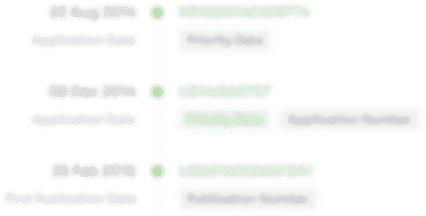
- R&D Engineer
- R&D Manager
- IP Professional
- Industry Leading Data Capabilities
- Powerful AI technology
- Patent DNA Extraction
Browse by: Latest US Patents, China's latest patents, Technical Efficacy Thesaurus, Application Domain, Technology Topic.
© 2024 PatSnap. All rights reserved.Legal|Privacy policy|Modern Slavery Act Transparency Statement|Sitemap