A classification and prediction method based on multi-stage hybrid model
A hybrid model and classification prediction technology, applied in genetic models, genetic rules, character and pattern recognition, etc., can solve problems such as long calculation time, ineffective research and exploration, and high computational complexity
- Summary
- Abstract
- Description
- Claims
- Application Information
AI Technical Summary
Problems solved by technology
Method used
Image
Examples
Embodiment 1
[0049] Embodiment 1, common multi-population niche genetic algorithm is exactly to add niche operation on multi-population genetic algorithm, concrete steps are as follows:
[0050] Step S1.1, initialization, generating a preset number of initial populations, and using the generated initial populations as the current population;
[0051] Step S1.2. For each current population, evaluate the population according to the fitness function corresponding to the candidate classifier;
[0052] Step S1.3, judging whether the termination condition of the iteration is satisfied, if it is satisfied, the iteration is ended and the optimal individual is output, otherwise, enter the next step;
[0053] Step S1.4, performing a selection operation on the current population;
[0054] Step S1.5, performing a cross operation on the current population;
[0055] Step S1.6, performing a mutation operation on the current population;
[0056] Step S1.7, perform niche operation on the current populatio...
Embodiment 2
[0059] Embodiment 2. Improved Multi-population Niche Genetic Algorithm. This embodiment combines multiple filtering methods to determine the importance of comprehensive features of all features. Based on the importance of comprehensive features, the original features are reordered, and according to their comprehensive Feature importance deletes some features in advance, and then uses the multi-population niche genetic algorithm to obtain the optimal feature subsets corresponding to different classifiers. The specific process is as figure 2 shown, including the following steps:
[0060] Step S2.1, using the hybrid filtering method to calculate the comprehensive feature importance of each feature, filter out the features whose comprehensive feature importance is not less than the set threshold, calculate the probability of the selected feature being selected according to the comprehensive feature importance, and generate a preset The number of initial populations, with the ini...
Embodiment 3
[0119] Embodiment 3, in the classifier selection stage, the multipopulation niche genetic algorithm adopted comprises the following steps:
[0120] Step 3.1. Obtain the probability of each candidate classifier being selected based on the predictive prior knowledge of the candidate classifiers, generate a preset number of initial populations, and use the initial population as the current population;
[0121] Step 3.2, use the corresponding fitness function to evaluate the population for each current population;
[0122] Step 3.3, judging whether the iteration termination condition is satisfied, if it is satisfied, then end the iteration and output the optimal individual, otherwise enter the next step;
[0123] Step 3.4, select the current population;
[0124] Step 3.5, perform cross operation on the current population;
[0125] Step 3.6, performing a mutation operation on the current population;
[0126] Step 3.7, carry out niche operation on the current population;
[0127...
PUM
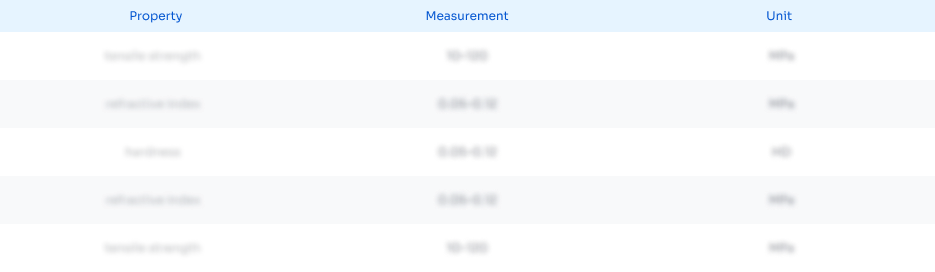
Abstract
Description
Claims
Application Information
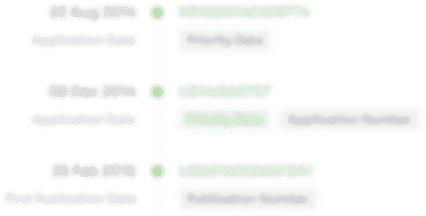
- R&D Engineer
- R&D Manager
- IP Professional
- Industry Leading Data Capabilities
- Powerful AI technology
- Patent DNA Extraction
Browse by: Latest US Patents, China's latest patents, Technical Efficacy Thesaurus, Application Domain, Technology Topic.
© 2024 PatSnap. All rights reserved.Legal|Privacy policy|Modern Slavery Act Transparency Statement|Sitemap