Photovoltaic Power Neural Network Prediction Method Based on Chaotic Phase Space Optimization and Reconstruction
A photovoltaic power generation and neural network technology, applied in the field of photovoltaic power generation neural network prediction method, can solve problems such as deviation accumulation, and achieve the effects of reducing prediction cost, avoiding deviation accumulation problems, and improving prediction accuracy and stability.
- Summary
- Abstract
- Description
- Claims
- Application Information
AI Technical Summary
Problems solved by technology
Method used
Image
Examples
Embodiment Construction
[0042] figure 1 Schematic flow chart of the neural network prediction method for photovoltaic power generation power reconstructed for chaotic phase space optimization, including the following steps:
[0043] (1) Use the improved C-C method to solve the optimal delay time τ and embedding dimension m of the preprocessed photovoltaic power time series, and perform phase space reconstruction;
[0044] (1.1) For the photovoltaic power generation time series p i (i=1,2...N), the associated integral defining the embedded time series is:
[0045]
[0046] Among them: N is the number of time series points, M is the number of points in each dimension in the reconstructed phase space, r is the defined space radius; H(a) is the step function, P(I), P(J) is the photovoltaic power time series Reconstructs two point phasors in phase space.
[0047] (1.2) Construction test statistics:
[0048] S 1 (m,N,r,τ)=C(m,N,r,τ)-C m (1,N,r,τ) (2)
[0049] In the process of calculating (2), if...
PUM
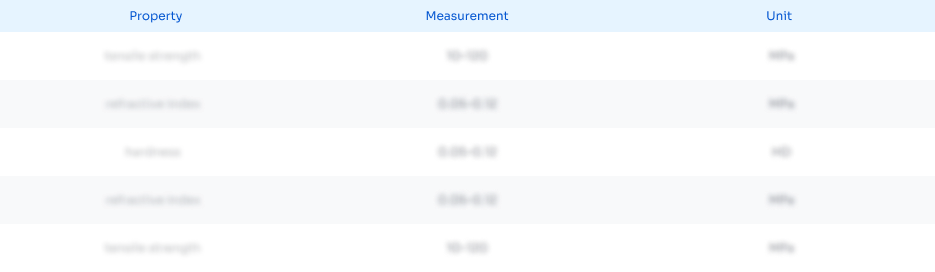
Abstract
Description
Claims
Application Information
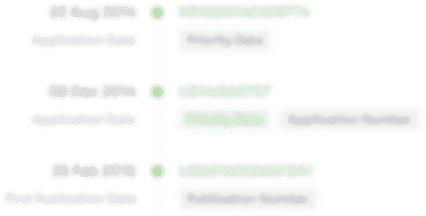
- R&D Engineer
- R&D Manager
- IP Professional
- Industry Leading Data Capabilities
- Powerful AI technology
- Patent DNA Extraction
Browse by: Latest US Patents, China's latest patents, Technical Efficacy Thesaurus, Application Domain, Technology Topic, Popular Technical Reports.
© 2024 PatSnap. All rights reserved.Legal|Privacy policy|Modern Slavery Act Transparency Statement|Sitemap|About US| Contact US: help@patsnap.com