An Android malware detection method based on depth learning
A malware and deep learning technology, applied in the field of computer and information science, which can solve the problems of low detection accuracy, difficulty in adapting to emerging software, and limited detection scope, and achieve high detection accuracy, easy adaptation, and enhanced features. learning effect
- Summary
- Abstract
- Description
- Claims
- Application Information
AI Technical Summary
Problems solved by technology
Method used
Image
Examples
Embodiment Construction
[0025] In order to better illustrate the purpose and advantages of the present invention, the implementation of the method of the present invention will be further described in detail below in conjunction with examples.
[0026] The specific process is:
[0027] Step 1, get Android positive and negative sample files, and then preprocess the files
[0028] In step 1.1, a total of 24,552 malicious Android software libraries were obtained from http: / / amd.arguslab.org / behaviors, and 21,000 normal software libraries were obtained from the Android market.
[0029] Step 1.2, for each application software, extract the AndroidManifest.xml file, res file, classes.dex file and other files of the Android application software through the Andguard tool for subsequent analysis.
[0030] Step 1.3, decompile the class.dex file through the Andguard tool, and then extract the Dalvik opcode of each Smalli file.
[0031] Step 2, feature extraction is performed on the Android application file.
...
PUM
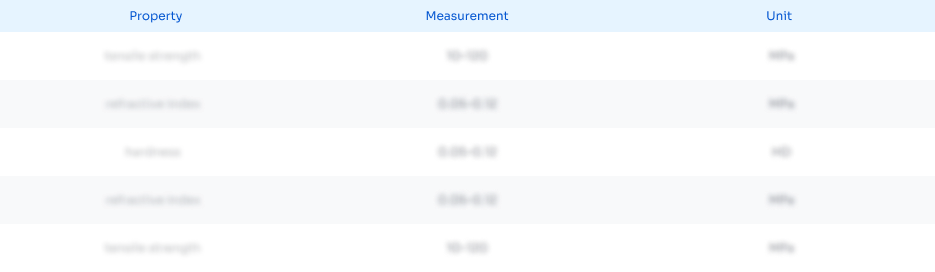
Abstract
Description
Claims
Application Information
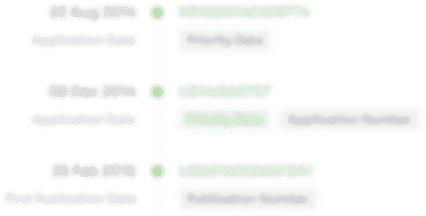
- Generate Ideas
- Intellectual Property
- Life Sciences
- Materials
- Tech Scout
- Unparalleled Data Quality
- Higher Quality Content
- 60% Fewer Hallucinations
Browse by: Latest US Patents, China's latest patents, Technical Efficacy Thesaurus, Application Domain, Technology Topic, Popular Technical Reports.
© 2025 PatSnap. All rights reserved.Legal|Privacy policy|Modern Slavery Act Transparency Statement|Sitemap|About US| Contact US: help@patsnap.com