Optical remote sensing image target detection method based on extended residual convolution
An optical remote sensing image and target detection technology, applied in the field of image processing, can solve the problems of large network feature receptive field, low resolution, large optical remote sensing image size, etc., and achieve the effect of improving detection accuracy and improving accuracy.
- Summary
- Abstract
- Description
- Claims
- Application Information
AI Technical Summary
Problems solved by technology
Method used
Image
Examples
Embodiment 1
[0032] In the prior art, due to the large size and low resolution of optical remote sensing images, especially in the detection of ships, the ship targets are small and the ship’s characteristics are often similar to those of some elongated buildings or large vehicle containers. As a result, this method often mistakenly detects objects on land as ships when detecting objects in optical remote sensing images. In view of this phenomenon, the present invention provides a deep convolutional network optical remote sensing image target detection method based on dilated residual convolution, see figure 1 The present invention uses expanded residual convolution and feature fusion in a deep convolutional network, and extracting features is more suitable for target detection tasks and can improve the accuracy of target detection in optical remote sensing images, including the following steps:
[0033] (1) Construct a test data set:
[0034] (1a) Use a window with a window size of 768×768×3 p...
Embodiment 2
[0052] The deep convolutional network optical remote sensing image target detection method based on dilated residual convolution is the same as that in Example 1-1. The test data set naming rule in step (1b) refers to each file to be cut for detecting optical remote sensing images The name and the corresponding windowing steps of the cutting data block are connected by the English underscore "_" symbol to form a .jpg file format.
Embodiment 3
[0054] The deep convolutional network optical remote sensing image target detection method based on dilated residual convolution is the same as in embodiment 1-2, the basic convolution module in step (3a) refers to: using 1×1 and 3×3 convolution kernels Build the basic convolution module, see figure 2 (a), the basic convolution module has three layers, and its block structure is: input layer → first convolution layer → second convolution layer → third convolution layer → output layer, input layer and output layer are cascaded →Final output layer.
[0055] The basic convolution module can be divided into four types according to the parameters of each convolution layer in the basic convolution module: basic convolution module one, basic convolution module two, basic convolution module three, and basic convolution module four.
[0056] The four basic convolution module parameters are as follows:
[0057] In the basic convolution module 1, the total number of feature maps of the first ...
PUM
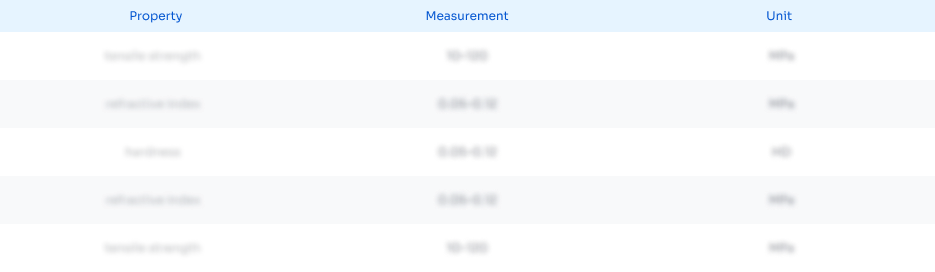
Abstract
Description
Claims
Application Information
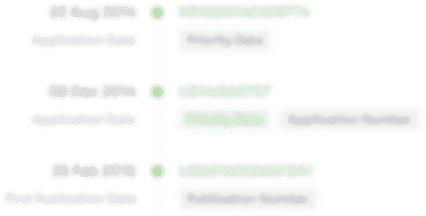
- R&D Engineer
- R&D Manager
- IP Professional
- Industry Leading Data Capabilities
- Powerful AI technology
- Patent DNA Extraction
Browse by: Latest US Patents, China's latest patents, Technical Efficacy Thesaurus, Application Domain, Technology Topic, Popular Technical Reports.
© 2024 PatSnap. All rights reserved.Legal|Privacy policy|Modern Slavery Act Transparency Statement|Sitemap|About US| Contact US: help@patsnap.com