A depth map super-resolution method
A super-resolution and depth map technology, applied in the fields of image processing and stereo vision, can solve the problems of depth map reconstruction interference and underutilization, and achieve the effect of sharp depth edge, suppressing ringing effect, and improving image resolution.
- Summary
- Abstract
- Description
- Claims
- Application Information
AI Technical Summary
Problems solved by technology
Method used
Image
Examples
Embodiment 1
[0036] The embodiment of the present invention proposes a color information-guided depth map super-resolution method, which uses a two-stream convolutional neural network structure to perform super-resolution on a low-resolution depth map, see figure 1 ,mainly include:
[0037] 101: Edge extraction of the depth image and the color image: that is, use the edge detection operator to extract the edge of the color image and the edge of the depth image after the initial upsampling;
[0038] 102: Optimization of the edge map, performing "AND" operation on the expanded color edge and depth edge;
[0039] 103: Super-resolution network construction, including four steps: image block extraction, feature map non-linear mapping, image reconstruction and optimized superposition.
[0040] Among them, super-resolution network training: driven by mean square error, stochastic gradient descent is the optimization strategy to update the learning parameters of the network.
[0041] To sum up,...
Embodiment 2
[0043] Combine below figure 1 and figure 2 The scheme in Example 1 is further introduced, see the following description for details:
[0044] 1. Edge extraction of depth map and color map
[0045] In order to make the edges of the depth map output by super-resolution sharper, it is necessary to use the outer edges of the color map in the same scene. However, since the resolutions of the high-resolution color image and the low-resolution depth map are different, first, the bicubic interpolation (Bicubic) algorithm is used to upsample the low-resolution depth map to make it the same size as the color map, and then use The edge detection operator (Canny) extracts the edges of depth images and color images:
[0046]
[0047] E. C =f c (Y)
[0048] in, Indicates that the low-resolution depth map D L Upsampling specific ratio, Y represents the color map, f c Indicates the edge extraction operator. E. C Represents the edge of the high-resolution color image obtained by...
Embodiment 3
[0084] Combine below figure 2 The scheme in embodiment 1 and 2 is carried out feasibility verification, see the following description for details:
[0085] figure 2 For the quantitative comparison of this method with other methods, the data are Teddy and Wood2 from the Middlebury stereo dataset.
[0086] Compared with the Bicubic method, the PSNR value obtained by this method can be increased by up to 26.10%. Since this method inputs the optimized edge map obtained from the color image and the depth image into the convolutional neural network, the optimized edge map guides the super-resolution reconstruction of the depth image, so the PSNR value of this method is also better than that of the SRCNN method. improvement.
[0087] Those skilled in the art can understand that the accompanying drawing is only a schematic diagram of a preferred embodiment, and the serial numbers of the above-mentioned embodiments of the present invention are for description only, and do not repr...
PUM
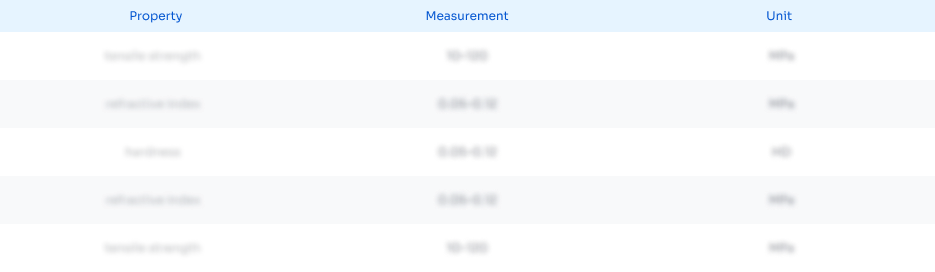
Abstract
Description
Claims
Application Information
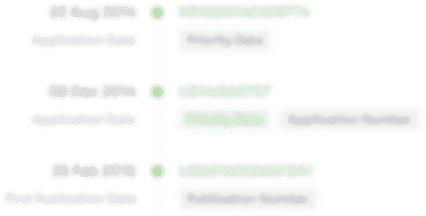
- R&D
- Intellectual Property
- Life Sciences
- Materials
- Tech Scout
- Unparalleled Data Quality
- Higher Quality Content
- 60% Fewer Hallucinations
Browse by: Latest US Patents, China's latest patents, Technical Efficacy Thesaurus, Application Domain, Technology Topic, Popular Technical Reports.
© 2025 PatSnap. All rights reserved.Legal|Privacy policy|Modern Slavery Act Transparency Statement|Sitemap|About US| Contact US: help@patsnap.com