OCT image quality evaluation method based on improved Resnet and SVR hybrid model
An image quality evaluation and hybrid model technology, applied in the field of medical image processing, can solve the problem of insufficient OCT image accuracy, and achieve the effect of avoiding information loss and small amount of data
- Summary
- Abstract
- Description
- Claims
- Application Information
AI Technical Summary
Problems solved by technology
Method used
Image
Examples
Embodiment 1
[0072] The present embodiment provides a kind of OCT image quality assessment method based on improved Resnet and SVR mixed model, comprises the following steps:
[0073] Step 1, preprocessing the original OCT image to obtain a preprocessed OCT image;
[0074] Since the original OCT image is different from the image finally displayed on the screen for the doctor to view, it needs to undergo gamma correction processing, and this embodiment evaluates the subjective perception quality of the OCT image, so it is necessary to perform gamma correction on the original OCT image ; On the other hand, due to the particularity of medical imaging, the data set used for training is small, which may lead to overfitting of the network. First, it is necessary to divide the corrected OCT image into blocks, so that the original OCT image The image becomes n OCT images, and n is the number of local OCT image blocks after block, which can make the effect of network training better. Therefore, th...
Embodiment 2
[0111] The difference between this embodiment and embodiment 1 is that in step 3, the deep-level features of the extracted preprocessed OCT image are used to train the OCT image quality evaluation model;
[0112] Given an OCT image to be evaluated, use the OCT image quality evaluation model to obtain the quality score of the OCT image to be evaluated;
[0113] Can also be:
[0114] Step 3.1, use the feature extraction network to extract the deep-level image features of each local OCT image block, and obtain the deep-level features of the OCT image through feature fusion;
[0115] In this embodiment, the last fully connected layer of the trained deep residual network is removed, and the remaining structure is used to extract deep image features of the input OCT image. The specific steps are as follows:
[0116] Step 3.1.1, remove the last fully connected layer of the deep residual network, at this time, the network input is the local OCT image block of m*m, and the output is t...
PUM
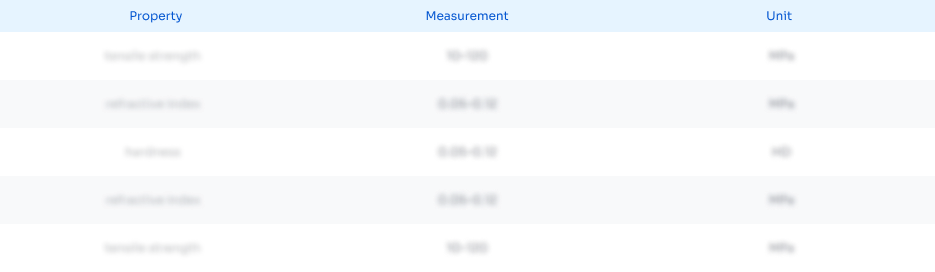
Abstract
Description
Claims
Application Information
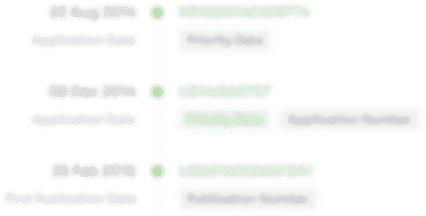
- Generate Ideas
- Intellectual Property
- Life Sciences
- Materials
- Tech Scout
- Unparalleled Data Quality
- Higher Quality Content
- 60% Fewer Hallucinations
Browse by: Latest US Patents, China's latest patents, Technical Efficacy Thesaurus, Application Domain, Technology Topic, Popular Technical Reports.
© 2025 PatSnap. All rights reserved.Legal|Privacy policy|Modern Slavery Act Transparency Statement|Sitemap|About US| Contact US: help@patsnap.com