A static image crowd counting method based on joint learning
A crowd counting and static image technology, applied in computing, computer components, instruments, etc., can solve the problems of insufficient data volume, poor model generalization ability, network overfitting and other problems in crowded scene datasets, and achieve the purpose of suppressing network overfitting. The effect of fitting problems, improving generalization ability, and high counting accuracy
- Summary
- Abstract
- Description
- Claims
- Application Information
AI Technical Summary
Problems solved by technology
Method used
Image
Examples
Embodiment
[0055] Shown in conjunction with the accompanying drawings is a specific implementation of the joint learning-based static image crowd counting method of the present invention, as shown in figure 1 As shown, the entire network framework includes a classifier (residual network) and three regressors (multi-column convolutional neural network). The purpose of the classifier is to automatically select the most suitable regressor for the image block, and the three regressors It is used to predict the crowd density map and then calculate the number of people.
[0056] The demonstration experiment of this embodiment uses two data sets: UCF_CC_50 and ShanghaiTech. The UCF_CC_50 dataset has 50 images of crowd scenes. The images of the dataset contain huge differences in the number of people. The minimum number of people in an image is 94, and the maximum number of people is 1279. The extremely small sample size and huge population variation make this dataset a very challenging one. T...
PUM
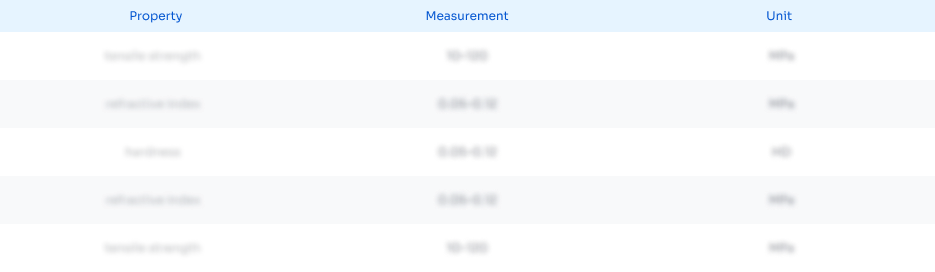
Abstract
Description
Claims
Application Information
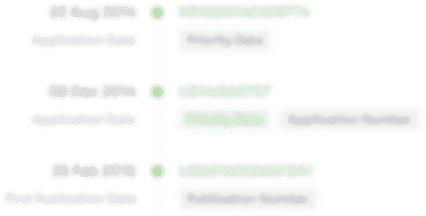
- R&D Engineer
- R&D Manager
- IP Professional
- Industry Leading Data Capabilities
- Powerful AI technology
- Patent DNA Extraction
Browse by: Latest US Patents, China's latest patents, Technical Efficacy Thesaurus, Application Domain, Technology Topic.
© 2024 PatSnap. All rights reserved.Legal|Privacy policy|Modern Slavery Act Transparency Statement|Sitemap