Deep-learning-based garbage identification evolutionary learning method, device, system and medium
A technology of deep learning and learning methods, applied in the field of garbage identification, can solve the problems of insufficient accuracy, reduce the workload of maintenance personnel, and identify the limited amount of garbage, so as to solve the problems of insufficient accuracy and quantity, human-computer interaction is helpful, Improving the effect of precision and breadth
- Summary
- Abstract
- Description
- Claims
- Application Information
AI Technical Summary
Problems solved by technology
Method used
Image
Examples
Embodiment 1
[0058] Machine learning is widely used in the field of object recognition, but most of its data sets rely on manpower, resulting in the trained model is always flawed, requiring high-frequency maintenance work by maintenance personnel. Therefore, this embodiment provides a garbage recognition evolutionary learning system based on deep learning. The system considers that in the field of garbage recognition, the types of garbage are changing with each passing day, and the data features that need to be extracted when the computer recognizes the target are also changeable, so the evolutionary learning system is proposed. The mechanism uses the data with poor recognition effect as the evolutionary power to update and improve the computer training model in real time to adapt to the changeable characteristics of the recognized garbage, and greatly improve the accuracy and breadth of recognition on the basis of existing technologies.
[0059] Such as figure 1 and figure 2 As shown, ...
Embodiment 2
[0077] Such as Figure 9 As shown, this embodiment implements the data processing of the information processor in the above-mentioned embodiment 1 through the server, that is, the server is directly connected to the camera and the controller. This embodiment provides a garbage recognition evolutionary learning method based on deep learning. The method Applied to the server, including the following steps:
[0078] S901. Obtain garbage sample image data.
[0079] S902. Perform preprocessing on the garbage sample image data, specifically: demeaning, normalization, PCA and whitening processing on the garbage sample image data.
[0080] S903. Using the preprocessed garbage sample image data as an input parameter of the neural network, compare it with the trained garbage recognition model, and judge whether the recognition is successful according to the comparison result, specifically:
[0081] The preprocessed garbage sample image data is used as the input parameter of the neural...
Embodiment 3
[0089] Such as Figure 10 As shown, this embodiment provides a garbage recognition evolutionary learning device based on deep learning, which includes an acquisition module 1001, a preprocessing module 1002, a first recognition module 1003, a feedback module 1004, a second recognition module 1005, a first The update module 1006 and the second update module 1007, the specific functions of each module are as follows:
[0090] The acquiring module 1001 is configured to acquire garbage sample image data.
[0091] The preprocessing module 1002 is used for preprocessing the garbage sample image data, specifically: for removing the mean value, normalizing, PCA and whitening the garbage sample image data.
[0092] The first recognition module 1003 is used to compare the preprocessed garbage sample image data with the trained garbage recognition model as the input parameter of the neural network, and judge whether the recognition is successful according to the comparison result, speci...
PUM
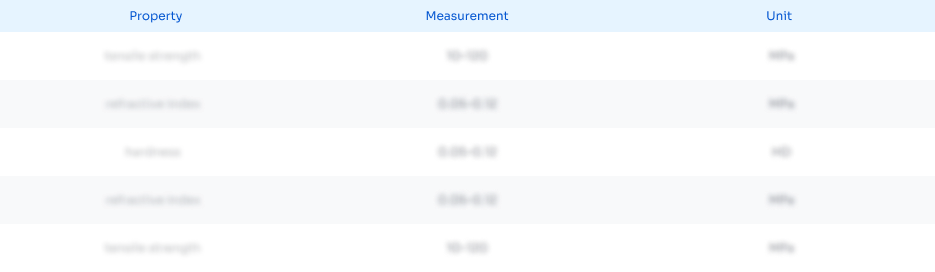
Abstract
Description
Claims
Application Information
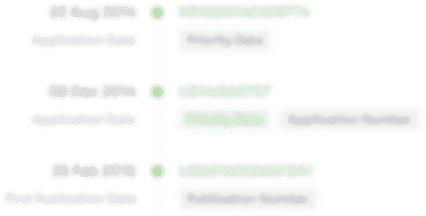
- Generate Ideas
- Intellectual Property
- Life Sciences
- Materials
- Tech Scout
- Unparalleled Data Quality
- Higher Quality Content
- 60% Fewer Hallucinations
Browse by: Latest US Patents, China's latest patents, Technical Efficacy Thesaurus, Application Domain, Technology Topic, Popular Technical Reports.
© 2025 PatSnap. All rights reserved.Legal|Privacy policy|Modern Slavery Act Transparency Statement|Sitemap|About US| Contact US: help@patsnap.com