A behavior recognition method of depth supervised convolution neural network based on training feature fusion
A convolutional neural network and feature fusion technology, applied in the field of artificial intelligence computer vision, can solve the problem of missing local information in video space
- Summary
- Abstract
- Description
- Claims
- Application Information
AI Technical Summary
Problems solved by technology
Method used
Image
Examples
Embodiment Construction
[0087] The specific implementation method of the present invention will be further described in detail below in conjunction with the accompanying drawings.
[0088]Execution environment of the present invention is to have computer to realize following three main functions to form: 1, multi-layer convolution feature extraction function, this function is to extract the multi-layer feature map of each frame of video. 2. Feature aggregation function, including the local evolution description pooling layer, the function of this layer is to encode the multi-frame feature map obtained by each layer into a local evolution descriptor; and the VLAD encoding layer based on the local evolution descriptor, the layer's The function is to encode local evolution descriptors into meta-action based video-level representations. 3. The method of deep supervised action recognition. The function of this method is to use the multi-layered video-level representation obtained above to identify the act...
PUM
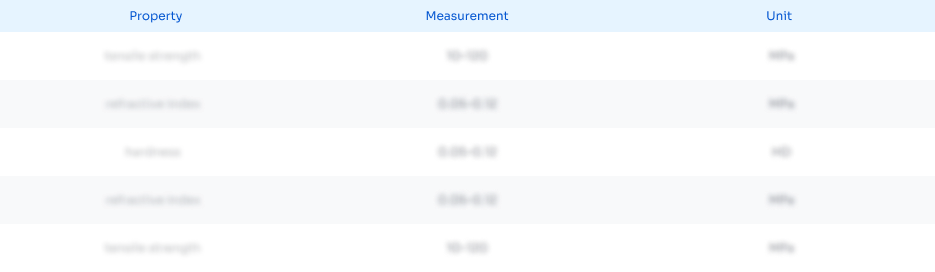
Abstract
Description
Claims
Application Information
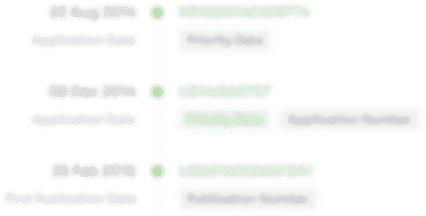
- R&D Engineer
- R&D Manager
- IP Professional
- Industry Leading Data Capabilities
- Powerful AI technology
- Patent DNA Extraction
Browse by: Latest US Patents, China's latest patents, Technical Efficacy Thesaurus, Application Domain, Technology Topic.
© 2024 PatSnap. All rights reserved.Legal|Privacy policy|Modern Slavery Act Transparency Statement|Sitemap