A support vector machine integrated learning method based on AdaBoost
A technology of support vector machine and ensemble learning, applied in the field of data mining and machine learning, which can solve problems such as low precision
- Summary
- Abstract
- Description
- Claims
- Application Information
AI Technical Summary
Problems solved by technology
Method used
Image
Examples
Embodiment 1
[0028] Embodiment 1: Take the state prediction of blast furnace temperature ([Si]) of Laiwu Iron & Steel No. 1 blast furnace (BF(a)) and Baotou No. 7 blast furnace (BF(b)) as an example. figure 2 The time series of blast furnace temperature [Si] and blast furnace air volume are given. Depend on figure 2 It can be seen that there are significant differences in scale between [Si] and air volume. Large-scale variables will cover up the impact of small-scale variables on the model, which will seriously affect the prediction accuracy of the model. To do this, first use the formula Normalize the sampled data so that the input variables are all on the same scale. Determine the training sample set and the test sample set, and conduct cluster analysis on the furnace temperature [Si] through the K-means algorithm on the training sample set, and divide the furnace temperature [Si] into three states: low temperature, high temperature and normal state. The low temperature and high t...
PUM
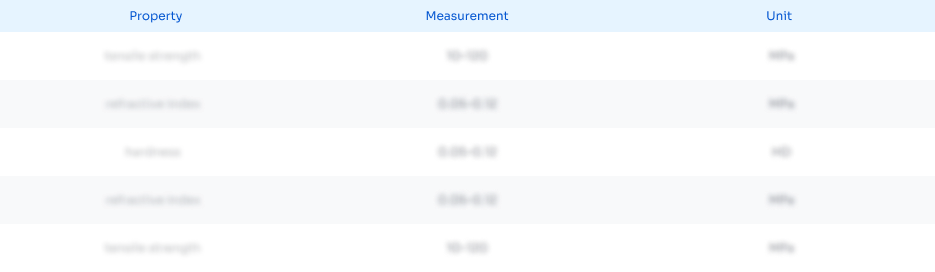
Abstract
Description
Claims
Application Information
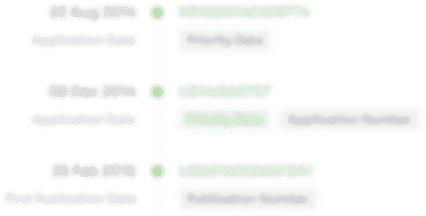
- R&D Engineer
- R&D Manager
- IP Professional
- Industry Leading Data Capabilities
- Powerful AI technology
- Patent DNA Extraction
Browse by: Latest US Patents, China's latest patents, Technical Efficacy Thesaurus, Application Domain, Technology Topic, Popular Technical Reports.
© 2024 PatSnap. All rights reserved.Legal|Privacy policy|Modern Slavery Act Transparency Statement|Sitemap|About US| Contact US: help@patsnap.com