Time-space domain correlation prediction method for air pollutant concentration
A technology of air pollutants and pollutant concentration, applied in prediction, biological neural network models, instruments, etc., can solve the problem of inability to realize deep connection extraction of data, limited ability to use large-scale data, and difficulty in temporal and spatial correlation of pollutants, etc. problem, to avoid the problem of gradient disappearance or gradient explosion, avoid the problem of gradient disappearance, and eliminate the effect of degradation problem
- Summary
- Abstract
- Description
- Claims
- Application Information
AI Technical Summary
Problems solved by technology
Method used
Image
Examples
Embodiment Construction
[0044] The present invention will be described in detail below with reference to the drawings and specific embodiments. This embodiment is implemented on the premise of the technical solution of the present invention, and a detailed implementation mode and specific operation process are given, but the protection scope of the present invention is not limited to the following embodiments.
[0045] This application first defines the air pollutant concentration prediction:
[0046] Definition 1 Air pollutant concentration prediction: It mainly uses historical pollutants and meteorological information to predict the concentration of a series of air pollution such as PM2.5 and PM10 in a certain period of time in the future. It is used in environmental science, meteorological science, computer science, etc. One of the key research topics, so it has a certain degree of interdisciplinary.
[0047] Definition 2 Traditional prediction method: Non-deep learning air pollutant concentration predi...
PUM
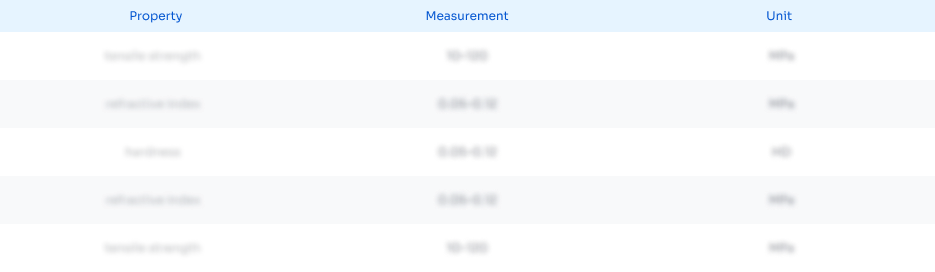
Abstract
Description
Claims
Application Information
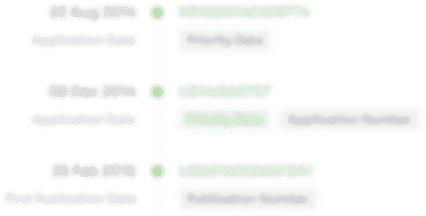
- R&D Engineer
- R&D Manager
- IP Professional
- Industry Leading Data Capabilities
- Powerful AI technology
- Patent DNA Extraction
Browse by: Latest US Patents, China's latest patents, Technical Efficacy Thesaurus, Application Domain, Technology Topic, Popular Technical Reports.
© 2024 PatSnap. All rights reserved.Legal|Privacy policy|Modern Slavery Act Transparency Statement|Sitemap|About US| Contact US: help@patsnap.com