Mobile pollution source emission concentration prediction method based on space-time deep learning
A technology of deep learning and emission concentration, applied in the direction of prediction, instrumentation, biological neural network model, etc., can solve the problem of low accuracy in predicting the concentration of air pollutants, and achieve high accuracy and stability
- Summary
- Abstract
- Description
- Claims
- Application Information
AI Technical Summary
Problems solved by technology
Method used
Image
Examples
Embodiment Construction
[0035] Such as figure 1 and figure 2 Shown, the present invention is concretely realized as follows:
[0036] Considering the spatio-temporal correlation among the 25 monitoring stations and the historical state of the monitoring stations, two or more CNN layers are selected to extract intrinsic features for long-term span learning from historical air pollutant data, followed by one-hot encoding. The method encodes hourly data and combines the extracted features with current weather data and associated pollutant data to improve the model's predictive performance. Two branches are used to extract spatial and temporal features, and then an attention model is used to weigh hidden features to enhance the effectiveness of features. By stacking multiple layers of LSTM, features of spatially correlated pollutant data with long-term dependencies can be automatically extracted layer by layer, and the fused features can be used to predict multi-scale time series forecasting of air po...
PUM
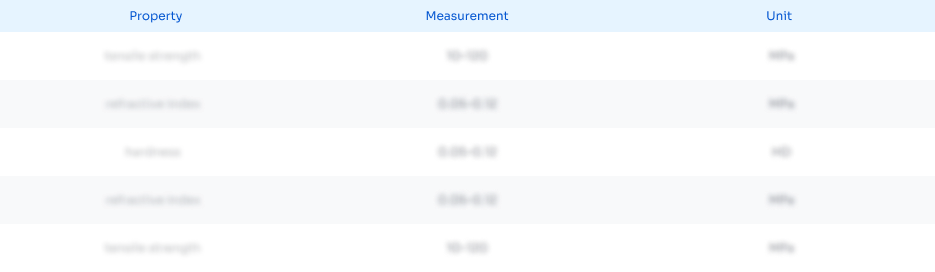
Abstract
Description
Claims
Application Information
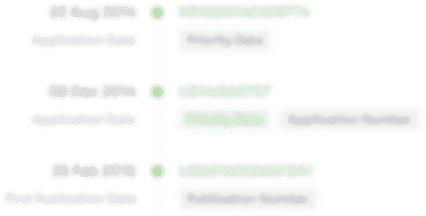
- R&D Engineer
- R&D Manager
- IP Professional
- Industry Leading Data Capabilities
- Powerful AI technology
- Patent DNA Extraction
Browse by: Latest US Patents, China's latest patents, Technical Efficacy Thesaurus, Application Domain, Technology Topic, Popular Technical Reports.
© 2024 PatSnap. All rights reserved.Legal|Privacy policy|Modern Slavery Act Transparency Statement|Sitemap|About US| Contact US: help@patsnap.com