Non-negative matrix factorization clustering method based on dual local learning
A clustering method and local learning technology, applied in the field of image processing, can solve the problem of high complexity of collaborative clustering
- Summary
- Abstract
- Description
- Claims
- Application Information
AI Technical Summary
Problems solved by technology
Method used
Image
Examples
Embodiment Construction
[0041] In order to more clearly illustrate the embodiments of the present invention or the technical solutions in the prior art, the specific implementation manners of the present invention will be described below with reference to the accompanying drawings. Obviously, the accompanying drawings in the following description are only some embodiments of the present invention, and those skilled in the art can obtain other accompanying drawings based on these drawings and obtain other implementations.
[0042] For non-negative matrix decomposition, the principle is: for any given non-negative data matrix V, by looking for a low rank, the non-negative original data matrix V is decomposed into a weight matrix X (matrix) and a feature matrix Y ( matrix) in the form of the product, so that the product of the two is approximately equal to the original data matrix as much as possible. Since the matrix before and after decomposition only contains non-negative elements, a column vector i...
PUM
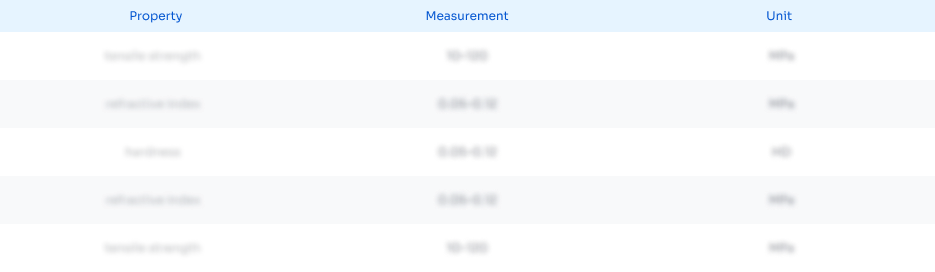
Abstract
Description
Claims
Application Information
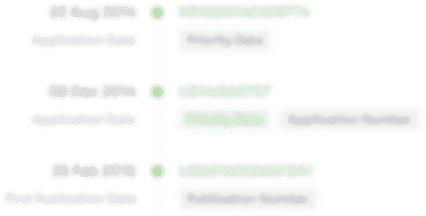
- R&D Engineer
- R&D Manager
- IP Professional
- Industry Leading Data Capabilities
- Powerful AI technology
- Patent DNA Extraction
Browse by: Latest US Patents, China's latest patents, Technical Efficacy Thesaurus, Application Domain, Technology Topic, Popular Technical Reports.
© 2024 PatSnap. All rights reserved.Legal|Privacy policy|Modern Slavery Act Transparency Statement|Sitemap|About US| Contact US: help@patsnap.com